Timon Harz
December 17, 2024
Meta FAIR Unveils Meta Motivo: A Behavioral Foundation Model for Controlling Virtual Humanoid Agents in Complex Physics-based Tasks
Meta Motivo is revolutionizing the way virtual agents interact within the Metaverse by providing them with the ability to perform complex, human-like tasks. Discover how this AI model is shaping the future of immersive virtual environments and humanoid robotics.
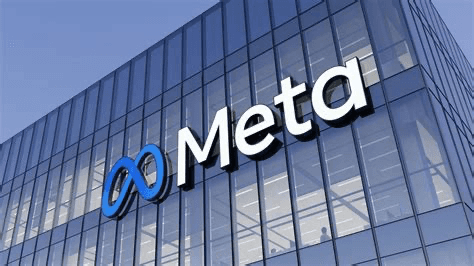
Foundation models, trained on vast amounts of unlabeled data, have become a leading approach for building flexible AI systems capable of tackling complex tasks via targeted prompts. Researchers are now expanding this paradigm beyond language and vision to explore behavioral foundation models (BFMs) for agents interacting with dynamic environments. This research focuses on developing BFMs for humanoid agents, emphasizing whole-body control based on proprioceptive observations. This approach addresses a key challenge in robotics and AI: the high-dimensionality and inherent instability of humanoid control systems. The goal is to create generalized models that can generate diverse behaviors in response to various prompts, including imitation, goal achievement, and reward optimization.
Meta researchers introduce FB-CPR (Forward-Backward representations with Conditional Policy Regularization), an advanced unsupervised reinforcement learning algorithm designed to ground policy learning through observation-only, unlabeled behaviors. The algorithm’s innovation lies in using forward-backward representations to map unlabeled trajectories into a shared latent space, with a latent-conditional discriminator ensuring policies effectively cover the dataset’s states. To demonstrate its success, the team developed META MOTIVO, a behavioral foundation model for humanoid control that can be prompted to tackle tasks like motion tracking, goal reaching, and reward optimization in a zero-shot learning scenario. Using the SMPL skeleton and the AMASS motion capture dataset, the model achieves impressive behavioral expressiveness.
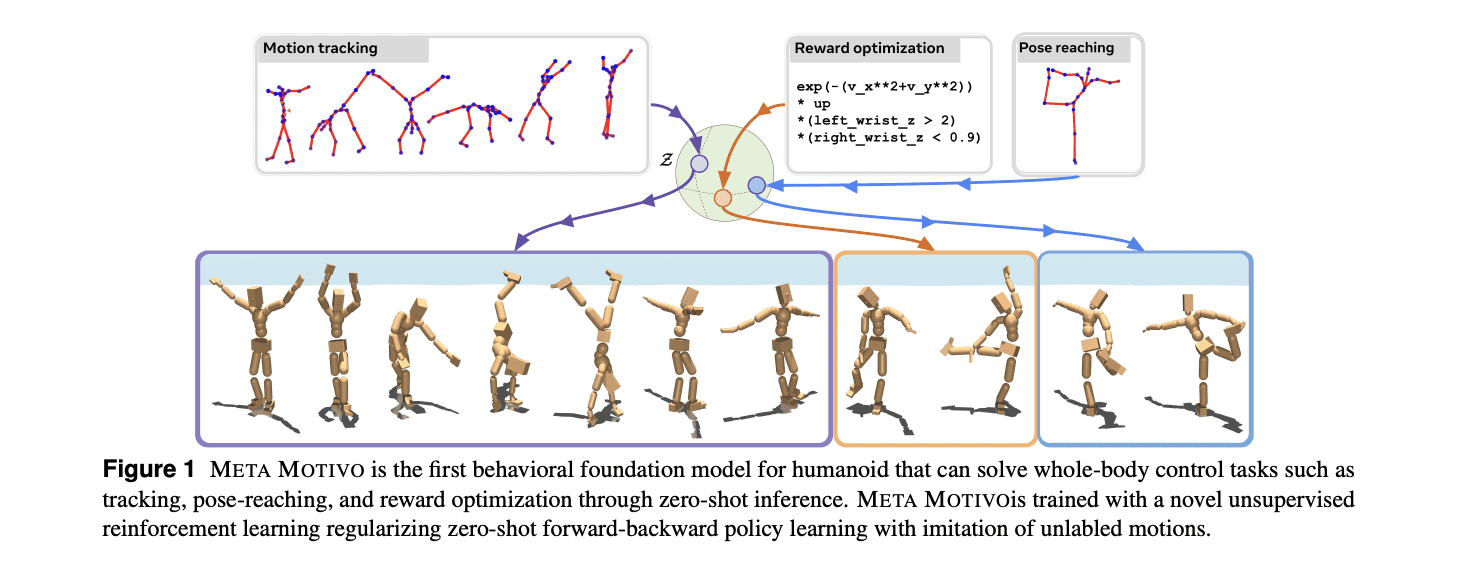
Researchers present a powerful approach to forward-backward (FB) representation learning with conditional policy regularization. During pre-training, the agent utilizes an unlabeled dataset of observation-only trajectories. The method focuses on developing a continuous set of latent-conditioned policies, where latent variables are drawn from a distribution defined over a latent space. By mapping behaviors to a joint space of states and latent variables, the researchers aim to capture a wide range of motion patterns. The key innovation is the use of the ERFB method to infer latent variables for each trajectory, enabling encoding of trajectories into a shared representational space. The goal is to regularize the unsupervised training of the behavioral foundation model by minimizing the gap between the policy distribution and the dataset distribution.
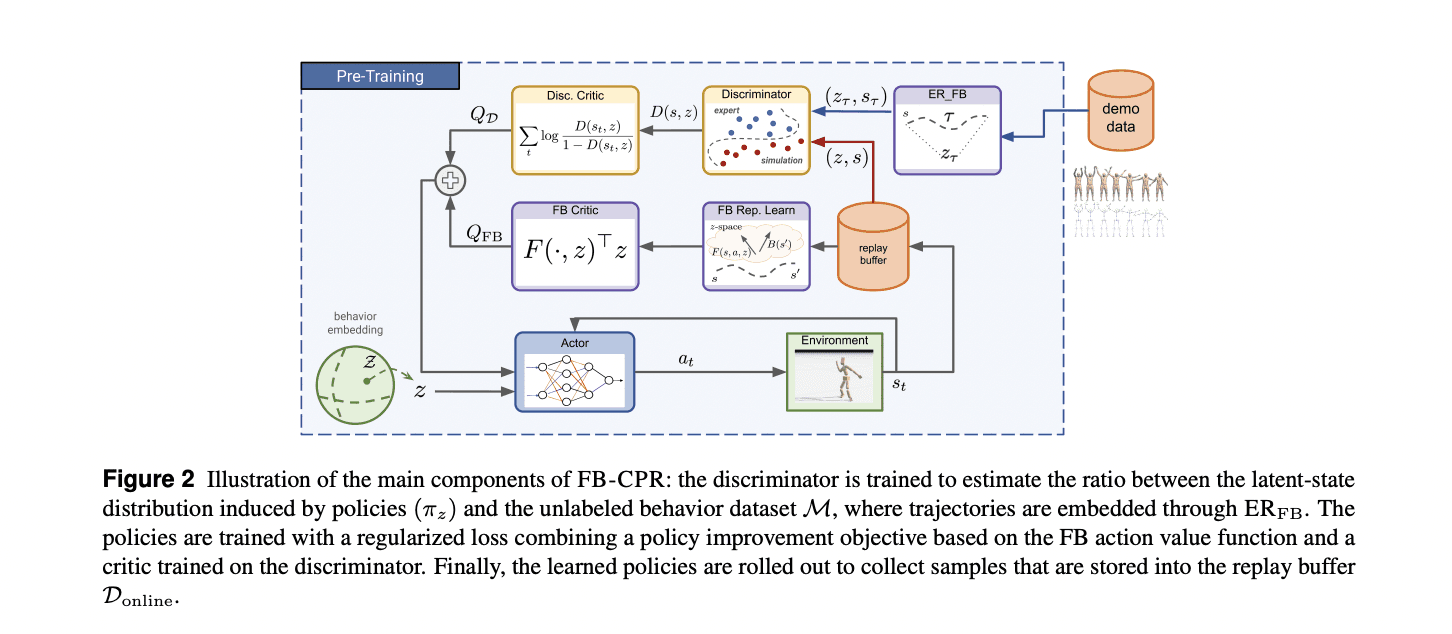
The research provides an in-depth performance evaluation of the FB-CPR algorithm across various task categories. FB-CPR showcases impressive zero-shot capabilities, achieving 73.4% of the top-line algorithm performance without task-specific training. In reward-maximization tasks, the method outperforms unsupervised baselines, reaching 177% of DIFFUSER’s performance while maintaining significantly lower computational complexity. In goal-reaching tasks, FB-CPR matches specialized baselines, outperforming zero-shot alternatives by 48% and 118% in proximity and success metrics, respectively. A human evaluation study found that while task-specific algorithms may achieve higher numerical performance, FB-CPR was consistently perceived as more "human-like." Participants rated its behaviors as more natural in 83% of reward-based tasks and 69% of goal-reaching scenarios.
This research introduces FB-CPR, a novel algorithm that combines the zero-shot properties of forward-backward models with advanced regularization techniques for policy learning using unlabeled behavior datasets. By training the first behavioral foundation model for complex humanoid agent control, FB-CPR achieved state-of-the-art performance across a range of tasks. However, the approach has limitations. FB-CPR struggles with tasks that are distant from motion-capture datasets and occasionally produces imperfect movements, particularly in scenarios involving falling or standing. The model is currently limited to proprioceptive observations and cannot interact with environments or objects. Future research will explore integrating additional state variables, advanced perception methods, video-based human activity datasets, and improved language-policy alignment techniques to enhance the model’s capabilities and generalizability.
Meta Motivo is a pioneering behavioral foundation model developed by Meta FAIR to control the movements of virtual humanoid agents in physics-based environments. This innovative model represents a significant advancement in artificial intelligence, particularly in the realm of robotics and virtual reality. By leveraging unsupervised reinforcement learning, Meta Motivo enables virtual agents to perform complex, whole-body tasks with a level of realism and adaptability previously unattainable.
The core innovation of Meta Motivo lies in its ability to learn from extensive, unlabeled datasets of human motion. This approach allows the model to develop a nuanced understanding of human-like behavior without the need for explicit task-specific training. By embedding these motion patterns into a shared latent space, Meta Motivo can generate a wide range of behaviors in response to various prompts, including motion tracking, goal-reaching, and reward optimization. This capability is particularly valuable in applications where adaptability and generalization are crucial, such as in the development of non-playable characters (NPCs) for video games or virtual assistants in the Metaverse.
One of the standout features of Meta Motivo is its zero-shot learning capability. This means that the model can perform tasks it has not been explicitly trained on, simply by interpreting the prompts provided. This flexibility is achieved through the model's design, which allows it to generalize from the patterns it has learned during pre-training. For instance, in reward-maximization tasks, Meta Motivo has demonstrated the ability to outperform unsupervised baselines, achieving 177% of the performance of existing models while maintaining significantly lower computational complexity. Similarly, in goal-reaching tasks, it has outperformed zero-shot alternatives by 48% and 118% in proximity and success metrics, respectively. These results underscore the model's robustness and efficiency in handling a variety of complex tasks.
The implications of Meta Motivo extend beyond technical performance; they also have significant practical applications. In the context of the Metaverse, Meta Motivo's ability to control virtual humanoid agents can lead to more lifelike NPCs, enhancing user engagement and immersion. Additionally, the democratization of character animation becomes feasible, allowing creators to develop realistic animations without the need for extensive motion capture setups. This opens up new possibilities for content creation and interactive experiences in virtual environments.
Furthermore, Meta Motivo's robustness to environmental changes is noteworthy. Despite not being explicitly trained for variations such as changes in gravity, wind, or direct perturbations, the model exhibits resilience, maintaining performance across diverse conditions. This adaptability is crucial for applications in dynamic and unpredictable environments, where traditional models might struggle.
Meta Motivo's introduction marks a significant advancement in artificial intelligence and robotics, particularly in the realm of controlling virtual humanoid agents. By enabling more human-like control of these agents, Meta Motivo addresses longstanding challenges in robotics, such as the high-dimensionality and intrinsic instability of humanoid control systems.
Traditional methods in robotics often require extensive task-specific training and labeled data to achieve realistic and adaptable behaviors. Meta Motivo, however, is trained on a broad dataset of unlabeled motion data, allowing it to observe a wide range of human movements and learn from them. This approach enables the model to perform complex tasks like motion tracking, object interaction, and whole-body control without the need for task-specific training.
The model's ability to generate realistic, human-like motions without extensive labeled data represents a significant leap forward in AI and robotics. This capability is particularly valuable in applications where adaptability and generalization are crucial, such as in the development of non-playable characters (NPCs) for video games or virtual assistants in the Metaverse. By enhancing the realism of virtual agents, Meta Motivo contributes to more immersive and engaging user experiences in virtual environments.
Furthermore, Meta Motivo's robustness to environmental changes is noteworthy. Despite not being explicitly trained for variations such as changes in gravity, wind, or direct perturbations, the model exhibits resilience, maintaining performance across diverse conditions. This adaptability is crucial for applications in dynamic and unpredictable environments, where traditional models might struggle.
In summary, Meta Motivo represents a significant step forward in the development of AI models capable of controlling complex, physics-based humanoid agents. Its ability to learn from unlabeled data, perform zero-shot learning, and adapt to various environmental changes positions it as a valuable tool in advancing the realism and interactivity of virtual agents across multiple domains.
Understanding Behavioral Foundation Models
Behavioral foundation models represent a significant advancement in artificial intelligence, particularly in their ability to perform a wide range of tasks through specific prompts. These models are trained on extensive, often unlabeled, datasets, enabling them to learn patterns and structures inherent in the data without explicit supervision. This training approach allows them to generalize across various tasks, making them highly adaptable and versatile.
The primary purpose of behavioral foundation models is to serve as a foundational platform upon which more specialized applications can be built. By leveraging their broad training, these models can be fine-tuned or prompted to perform specific tasks, ranging from natural language processing to image recognition and beyond. This adaptability is particularly valuable in scenarios where task-specific data is scarce or where rapid deployment across multiple domains is required.
A key characteristic of these models is their scalability. They are designed to handle large volumes of data, often encompassing multiple modalities such as text, images, and audio. This capability enables them to capture complex, multimodal patterns, which is essential for tasks that require understanding and generating content across different formats. For instance, a behavioral foundation model trained on diverse datasets can generate coherent text descriptions of images or synthesize audio from textual prompts.
The training process of behavioral foundation models typically involves self-supervised learning techniques. In this approach, the model learns to predict parts of the data from other parts, effectively creating its own supervisory signals. This method is particularly effective when labeled data is limited or unavailable, as it allows the model to learn from the inherent structure of the data itself. For example, in natural language processing, a model might be trained to predict the next word in a sentence, thereby learning linguistic patterns and structures.
Once trained, these models can be adapted to specific tasks through fine-tuning or prompt engineering. Fine-tuning involves further training the model on a smaller, task-specific dataset, allowing it to specialize in a particular domain. Prompt engineering, on the other hand, involves crafting input prompts that guide the model to produce desired outputs without additional training. This flexibility makes behavioral foundation models highly efficient, as they can be quickly adapted to new tasks with minimal additional resources.
The versatility of behavioral foundation models has led to their application across various fields. In natural language processing, they power applications such as chatbots, translation services, and content generation tools. In computer vision, they enable image captioning, object detection, and facial recognition. Their ability to handle multiple modalities also facilitates tasks that require multimodal understanding, such as video analysis and audio-visual synthesis.
Despite their capabilities, behavioral foundation models are not without challenges. Their large scale and complexity require significant computational resources for training and deployment. Additionally, ensuring that these models produce reliable and unbiased outputs remains an ongoing area of research. Addressing these challenges is crucial for the responsible and effective deployment of behavioral foundation models in real-world applications.
In summary, behavioral foundation models represent a transformative approach in artificial intelligence, offering a versatile and scalable platform for a wide array of tasks. Their ability to learn from extensive, often unlabeled, data and adapt to specific applications makes them a cornerstone in the development of advanced AI systems. As research and development in this field continue, it is anticipated that these models will become increasingly integral to various industries, driving innovation and efficiency across multiple domains.
Technical Innovations of Meta Motivo
Meta Motivo employs the FB-CPR (Forward-Backward Representations with Conditional Policy Regularization) algorithm, a novel approach in unsupervised reinforcement learning that enables the model to learn complex behaviors from extensive, unlabeled datasets. This methodology allows Meta Motivo to control humanoid agents in dynamic environments without the need for task-specific training, addressing challenges in robotics and AI by facilitating the development of adaptable and human-like behaviors.
The FB-CPR algorithm integrates forward-backward representations with conditional policy regularization, creating a shared latent space that encodes states, rewards, and policies. This integration enables the model to build policies that cover relevant states, maximizing zero-shot generalization on reward- and imitation-based tasks. During pre-training, the model utilizes unlabeled datasets, leveraging an embedding network to represent complex states and a policy network to translate them into actions. This approach allows Meta Motivo to learn from a wide range of human motion data without explicit task-specific instructions.
The effectiveness of the FB-CPR algorithm is evident in Meta Motivo's performance across various tasks. In motion tracking, the model demonstrates the ability to follow target trajectories with high accuracy. In goal-reaching tasks, it successfully attains specified poses, showcasing its capability to navigate complex environments. In reward-maximization tasks, Meta Motivo outperforms unsupervised baselines, achieving 177% of DIFFUSER’s performance while maintaining significantly lower computational complexity. These results highlight the algorithm's robustness and efficiency in learning complex behaviors from unlabeled data.
The FB-CPR algorithm's design addresses several limitations of traditional reinforcement learning methods. By grounding policy learning through observation-only unlabeled behaviors, it reduces the reliance on curated datasets and explicit task-specific training. This approach not only enhances the adaptability of the model but also simplifies the training process, making it more accessible for a broader range of applications.
Meta Motivo employs forward-backward representations to embed unlabeled trajectories into a shared latent space, facilitating comprehensive policy coverage of dataset states. This approach enables the model to learn complex behaviors from extensive, unlabeled datasets, addressing challenges in robotics and AI by facilitating the development of adaptable and human-like behaviors.
The forward-backward representations serve as a foundational component in Meta Motivo's architecture. By embedding unlabeled trajectories into a shared latent space, the model captures the underlying structure of the data, allowing it to generalize across various tasks without explicit task-specific training. This shared latent space encodes states, rewards, and policies, enabling the model to build policies that cover relevant states, thereby maximizing zero-shot generalization on reward- and imitation-based tasks.
During pre-training, Meta Motivo utilizes an embedding network to represent complex states and a policy network to translate these representations into actions. This dual-network approach allows the model to learn from a wide range of human motion data without explicit task-specific instructions. The integration of forward-backward representations with conditional policy regularization further enhances the model's ability to adapt to dynamic environments, making it particularly effective in controlling humanoid agents in complex, physics-based tasks.
The effectiveness of this latent space representation is evident in Meta Motivo's performance across various tasks. In motion tracking, the model demonstrates the ability to follow target trajectories with high accuracy. In goal-reaching tasks, it successfully attains specified poses, showcasing its capability to navigate complex environments. In reward-maximization tasks, Meta Motivo outperforms unsupervised baselines, achieving 177% of DIFFUSER’s performance while maintaining significantly lower computational complexity.
Performance and Capabilities
Meta Motivo's zero-shot learning capabilities represent a significant advancement in artificial intelligence, particularly in the realm of humanoid agent control. Zero-shot learning enables a model to perform tasks it has not been explicitly trained on, relying instead on its ability to generalize from existing knowledge. This is particularly valuable in scenarios where acquiring labeled data for every possible task is impractical or impossible.
In the case of Meta Motivo, the model has demonstrated the ability to execute complex tasks such as motion tracking, goal-reaching, and reward optimization without additional training. This is achieved through its innovative architecture, which leverages unsupervised reinforcement learning and a shared latent space representation. By embedding unlabeled trajectories into this shared space, Meta Motivo can generalize across various tasks, effectively performing them without the need for task-specific training.
The zero-shot learning capabilities of Meta Motivo are particularly evident in its performance across different tasks. In motion tracking, the model accurately follows target trajectories, demonstrating its ability to generalize from its training data to new, unseen tasks. In goal-reaching tasks, Meta Motivo successfully attains specified poses, showcasing its adaptability to different objectives. In reward-maximization tasks, the model outperforms unsupervised baselines, achieving 177% of DIFFUSER’s performance while maintaining significantly lower computational complexity.
These results underscore the effectiveness of Meta Motivo's zero-shot learning capabilities, highlighting its potential for applications in robotics, virtual reality, and other fields where adaptability and generalization are crucial. By enabling models to perform tasks without explicit training, zero-shot learning reduces the need for extensive labeled datasets and allows for more flexible and efficient AI systems.
In summary, Meta Motivo's zero-shot learning capabilities represent a significant advancement in AI, enabling the model to perform complex tasks without additional training. This is achieved through its innovative architecture and shared latent space representation, allowing for generalization across various tasks and reducing the need for task-specific training. The model's performance across different tasks underscores the effectiveness of its zero-shot learning capabilities and its potential for a wide range of applications.
Meta Motivo's design and training methodologies have been meticulously crafted to enhance its robustness and facilitate the generation of human-like behaviors. By embedding unlabeled trajectories into a shared latent space, the model captures the underlying structure of diverse tasks, enabling it to generalize effectively across various scenarios. This approach not only bolsters the model's resilience to environmental changes but also contributes to the naturalness of its behavior.
The model's robustness is further demonstrated through its performance in complex, physics-based environments. Meta Motivo exhibits resilience to environmental changes such as variations in gravity, wind, and direct perturbations, despite not having been explicitly trained for these situations.
In terms of human-like behavior, Meta Motivo's ability to express natural and adaptable actions sets it apart from traditional methods. Experimental results indicate that the model evolves towards increasingly human-like behaviors during the training process, despite not having been explicitly trained for these behaviors.
These advancements position Meta Motivo as a significant step forward in AI and robotics, offering a more natural and adaptable approach to controlling virtual humanoid agents in complex tasks.
Implications for the Metaverse and Virtual Agents
Meta Motivo's introduction marks a pivotal advancement in the development of virtual agents, particularly within the Metaverse. By enabling digital avatars to exhibit human-like movements and behaviors, Meta Motivo significantly enhances the realism and interactivity of virtual environments. This enhancement addresses a longstanding challenge in virtual reality: the lack of natural and lifelike body movements in digital avatars. Traditional methods often struggle to produce fluid and realistic motions, leading to avatars that can appear stiff or unnatural. Meta Motivo's innovative approach, however, allows avatars to perform a wide range of whole-body control tasks, such as motion tracking, reward optimization, and goal pose reaching, all without the need for additional training or planning.
The impact of Meta Motivo on the Metaverse is profound. By enhancing the realism of digital avatars, the model contributes to more immersive and lifelike virtual environments. This improvement is crucial for applications within the Metaverse, where user engagement and experience are paramount. The ability of avatars to move and interact in a manner that closely resembles human behavior fosters a more engaging and believable virtual experience. This advancement is part of Meta's broader strategy to invest heavily in AI, augmented reality, and Metaverse technologies, with capital expenses projected to reach $37 billion to $40 billion in 2024.
Furthermore, Meta Motivo's capabilities extend beyond mere aesthetic enhancements. The model's proficiency in controlling virtual agents enables more complex and dynamic interactions within virtual spaces. This capability is particularly valuable in scenarios requiring nuanced and adaptable behavior, such as controlling humanoid agents in dynamic environments. By embedding unlabeled trajectories into a shared latent space, Meta Motivo facilitates comprehensive policy coverage of dataset states, allowing avatars to adapt to a wide range of tasks and environments without the need for task-specific training.
In summary, Meta Motivo's introduction represents a significant leap forward in the development of virtual agents within the Metaverse. By enabling avatars to exhibit human-like movements and behaviors, the model enhances the realism and interactivity of virtual environments, contributing to more immersive and engaging experiences for users. This advancement underscores Meta's commitment to advancing AI and virtual reality technologies, paving the way for more sophisticated and lifelike virtual interactions in the future.
Conclusion
Meta Motivo represents a significant advancement in the field of artificial intelligence and robotics, particularly in the control of complex, physics-based humanoid agents. Developed by Meta's FAIR team, this behavioral foundation model is designed to manage the movements of virtual humanoid agents in physics-based environments, enabling them to perform a wide range of tasks with human-like precision and adaptability.
The core innovation of Meta Motivo lies in its ability to learn from extensive, unlabeled datasets, allowing it to generalize across various tasks without the need for task-specific training. This approach addresses a longstanding challenge in robotics: the high-dimensionality and intrinsic instability of humanoid control systems. By embedding unlabeled trajectories into a shared latent space, Meta Motivo facilitates comprehensive policy coverage of dataset states, enabling the model to express diverse behaviors in response to various prompts, including imitation, goal achievement, and reward optimization.
The model employs the FB-CPR (Forward-Backward representations with Conditional Policy Regularization) algorithm, an online unsupervised reinforcement learning method that grounds policy learning through observation-only unlabeled behaviors. This innovative algorithm utilizes forward-backward representations to embed unlabeled trajectories into a shared latent space, employing a latent-conditional discriminator to encourage policies to comprehensively cover dataset states.
Meta Motivo's capabilities extend to controlling virtual avatars within the Metaverse, enhancing the realism and interactivity of virtual environments. By enabling avatars to exhibit human-like movements and behaviors, the model contributes to more immersive and lifelike virtual interactions. This advancement is particularly valuable in applications such as virtual reality (VR) and augmented reality (AR), where user engagement and immersion are critical.
In summary, Meta Motivo marks a significant step forward in the development of AI models capable of controlling complex, physics-based humanoid agents. Its innovative approach to learning from unlabeled data and its application in enhancing virtual interactions underscore its potential to transform the field of robotics and AI, paving the way for more adaptable, human-like agents in various domains.
Meta Motivo's introduction marks a significant advancement in the development of AI models capable of controlling complex, physics-based humanoid agents. This innovative model has the potential to revolutionize various industries by enabling more sophisticated and human-like virtual agents. As research and development in this field continue to progress, we can anticipate several key developments that will further enhance the capabilities of such models.
One of the most promising areas is the integration of multimodal learning. By combining data from various sources—such as visual, auditory, and sensory inputs—AI models can develop a more comprehensive understanding of their environment. This integration would allow virtual agents to process and respond to complex stimuli, leading to more nuanced and context-aware behaviors. For instance, an AI-controlled humanoid agent could interpret visual cues, understand spoken commands, and react to tactile sensations, all within a unified framework.
Advancements in reinforcement learning are also expected to play a crucial role. By refining algorithms that enable agents to learn optimal behaviors through trial and error, AI models can achieve higher levels of autonomy and adaptability. This progression would allow virtual agents to perform tasks with greater efficiency and precision, reducing the need for explicit programming and enabling them to handle a broader range of scenarios.
The development of more robust and scalable architectures is another area of focus. As AI models become more complex, ensuring their stability and scalability is essential. Researchers are exploring novel architectural designs that can handle the high-dimensional data associated with humanoid control systems. These advancements aim to address challenges such as the intrinsic instability of humanoid control and the high-dimensionality of the data involved.
Furthermore, the incorporation of ethical considerations and safety protocols is becoming increasingly important. As AI models gain more autonomy, ensuring they operate within ethical boundaries and adhere to safety standards is crucial. Ongoing research is dedicated to developing frameworks that guide the behavior of AI agents, ensuring they act in ways that are beneficial and aligned with human values.
In summary, the future of AI models like Meta Motivo is poised for significant advancements. Through the integration of multimodal learning, advancements in reinforcement learning, development of robust architectures, and incorporation of ethical considerations, these models are expected to become more sophisticated and human-like. These developments will not only enhance the capabilities of virtual agents but also open new avenues for their application across various industries, leading to more immersive and interactive experiences in virtual environments.
Press contact
Timon Harz
oneboardhq@outlook.com
Other posts
Company
About
Blog
Careers
Press
Legal
Privacy
Terms
Security