Timon Harz
December 17, 2024
Meta AI Launches Apollo: A New Family of Video LLMs for Advanced Video Understanding and Multimodal Applications
Meta AI's Apollo is setting new standards for video comprehension with its ability to analyze complex video data. Learn how this cutting-edge model is transforming industries and driving innovation in multimodal AI applications.
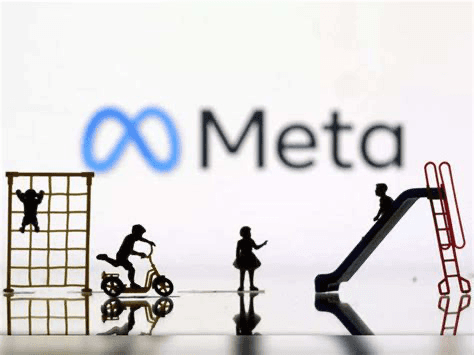
While multimodal models (LMMs) have made significant strides in text and image processing, video-based models are still lagging behind. Videos are inherently complex, combining both spatial and temporal elements, which require substantial computational resources. Current approaches often adapt image-based models or rely on uniform frame sampling, failing to capture the full scope of motion and temporal relationships. Additionally, training large-scale video models is computationally intensive, making it difficult to explore different design approaches effectively.
To address these challenges, researchers from Meta AI and Stanford have developed Apollo, a new family of video-focused LMMs aimed at advancing video understanding. Apollo overcomes these issues through innovative design choices that enhance efficiency and set a new standard for tasks such as temporal reasoning and video-based question answering.
Meta AI Introduces Apollo: A Family of Scalable Video-LMMs
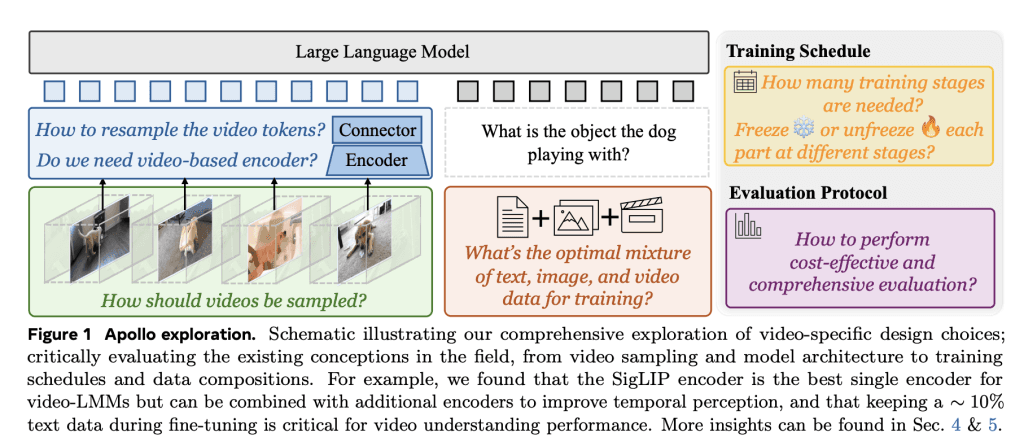
Meta AI's Apollo models are built to handle videos up to an hour long while delivering strong performance across key video-language tasks. Available in three sizes—1.5B, 3B, and 7B parameters—Apollo offers flexibility to meet various computational requirements and practical applications.
Key innovations include:
Scaling Consistency: Design choices made in smaller models are successfully transferred to larger versions, minimizing the need for extensive large-scale experiments.
Frame-Per-Second (fps) Sampling: A more efficient sampling technique than uniform frame sampling, ensuring improved temporal consistency in video processing.
Dual Vision Encoders: Combining SigLIP for spatial understanding and InternVideo2 for temporal reasoning, Apollo achieves a well-balanced representation of video data.
ApolloBench: A curated benchmark suite that reduces redundancy in evaluation while providing in-depth insights into model performance.
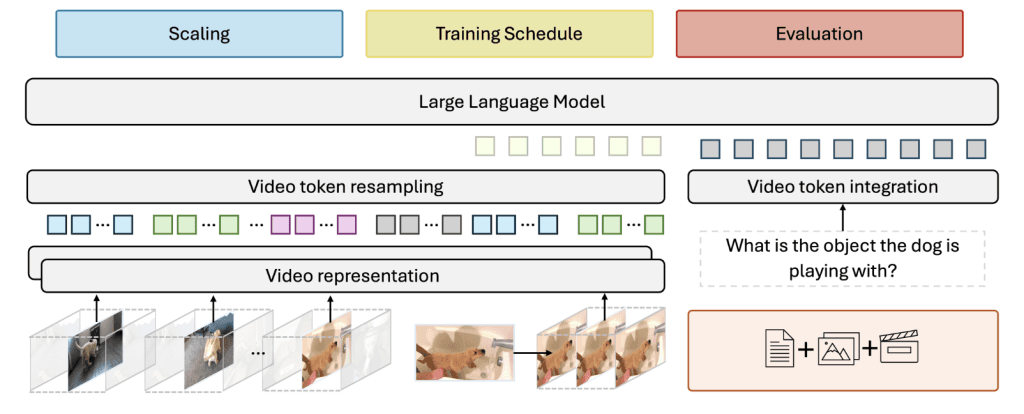
Technical Highlights and Advantages
The Apollo models are designed with several well-researched innovations aimed at addressing the challenges inherent in video-based LMMs:
Frame-Per-Second (fps) Sampling: Unlike traditional uniform frame sampling, Apollo’s fps sampling technique ensures temporal consistency, allowing the models to more effectively understand motion, speed, and the sequence of events in videos.
Scaling Consistency: Apollo’s design choices for smaller models (2B-4B parameters) generalize effectively to larger models, which reduces the need for costly large-scale experiments and minimizes computational resources without sacrificing performance.
Dual Vision Encoders: Apollo integrates two complementary encoders—SigLIP for spatial understanding and InternVideo2 for temporal reasoning—working together to create more accurate and nuanced video representations.
Token Resampling: Apollo uses a Perceiver Resampler to efficiently reduce video tokens without sacrificing critical information, enabling the model to process long videos with minimal computational overhead.
Optimized Training: The Apollo training process follows a three-stage approach, fine-tuning video encoders on video data first, before integrating text and image datasets. This ensures stable and effective learning for video-based tasks.
Multi-Turn Conversations: Apollo supports interactive, multi-turn conversations grounded in video content, making it ideal for applications like video-based chat systems and content analysis.
Performance Insights
Apollo's performance is validated through impressive benchmark results, frequently surpassing even larger models:
Apollo-1.5B:
Outperforms models like Phi-3.5-Vision (4.2B) and LongVA-7B.
Scores: 60.8 on Video-MME, 63.3 on MLVU, 57.0 on ApolloBench.
Apollo-3B:
Competes with and outperforms several 7B models.
Scores: 58.4 on Video-MME, 68.7 on MLVU, 62.7 on ApolloBench.
Achieves 55.1 on LongVideoBench.
Apollo-7B:
Matches or even surpasses models with over 30B parameters, including Oryx-34B and VILA1.5-40B.
Scores: 61.2 on Video-MME, 70.9 on MLVU, 66.3 on ApolloBench.
Benchmark Summary:
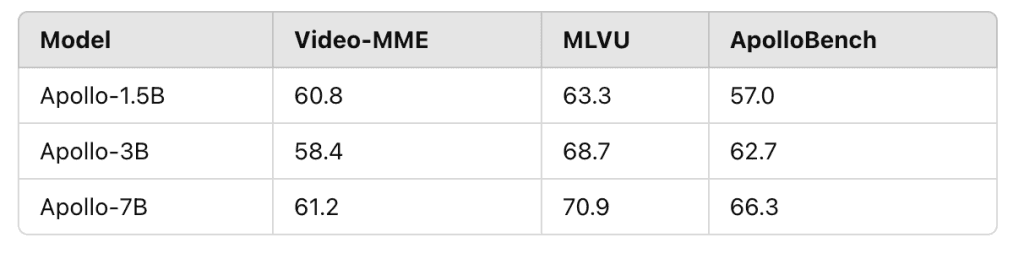
Apollo represents a major advancement in the development of video-based LMMs. By tackling critical challenges like efficient video sampling and model scalability, Apollo delivers a robust and effective solution for understanding video content. Its ability to outperform larger models underscores the value of thoughtful design and strategic training approaches.
The Apollo family presents practical solutions for a wide range of real-world applications, including video-based question answering, content analysis, and interactive systems. Additionally, Meta AI’s introduction of ApolloBench offers a more streamlined and efficient benchmark for evaluating video-LMMs, setting the stage for future research and innovation in the field.
Meta AI has recently unveiled Apollo, a groundbreaking family of Large Multimodal Models (LLMs) specifically engineered to advance video understanding and support a wide array of multimodal applications. This launch signifies a pivotal moment in artificial intelligence, addressing the intricate challenges associated with processing and interpreting video content.
Addressing the Challenges of Video Understanding
Videos inherently present complexities due to their combination of spatial and temporal dimensions. Traditional AI models often struggle to effectively capture the nuances of motion, sequence, and context within video data. Existing methods frequently adapt image-based approaches or rely on uniform frame sampling, which can inadequately represent the dynamic nature of video content. Moreover, training large-scale video models demands substantial computational resources, making it challenging to explore design choices efficiently.
Innovative Design and Features of Apollo
Apollo addresses these challenges through several innovative design choices:
Frame-Per-Second (fps) Sampling: Apollo employs a more efficient video sampling technique compared to uniform frame sampling, ensuring better temporal consistency.
Dual Vision Encoders: By combining SigLIP for spatial understanding with InternVideo2 for temporal reasoning, Apollo enables a balanced representation of video data.
Scaling Consistency: Design choices made on smaller models are shown to transfer effectively to larger ones, reducing the need for large-scale experiments.
Token Resampling: Utilizing a Perceiver Resampler, Apollo efficiently reduces video tokens without losing information, allowing the models to process long videos without excessive computational overhead.
Optimized Training: Apollo employs a three-stage training process where video encoders are initially fine-tuned on video data before integrating with text and image datasets. This staged approach ensures stable and effective learning.
Multi-Turn Conversations: Apollo models can support interactive, multi-turn conversations grounded in video content, making them ideal for applications like video-based chat systems or content analysis.
Performance and Benchmarks
Apollo's performance has been rigorously evaluated across multiple benchmarks, often surpassing larger models:
Apollo-1.5B: Surpasses models like Phi-3.5-Vision (4.2B) and LongVA-7B, achieving scores of 60.8 on Video-MME, 63.3 on MLVU, and 57.0 on ApolloBench.
Apollo-3B: Competes with and outperforms many 7B models, with scores of 58.4 on Video-MME, 68.7 on MLVU, and 62.7 on ApolloBench. It also achieves 55.1 on LongVideoBench.
Apollo-7B: Matches and even surpasses models with over 30B parameters, such as Oryx-34B and VILA1.5-40B, with scores of 61.2 on Video-MME, 70.9 on MLVU, and 66.3 on ApolloBench.
Implications for Multimodal Applications
The introduction of Apollo holds significant implications for various sectors:
Enhanced Video Analysis: Apollo's capabilities enable more accurate and efficient video analysis, benefiting sectors like entertainment, security, and education.
Integration with Multimodal Systems: The models can be integrated into systems requiring the processing of multiple data types, such as virtual assistants and autonomous vehicles.
Meta AI's introduction of Apollo marks a significant milestone in the evolution of artificial intelligence, particularly in the realm of video understanding and multimodal applications. This development addresses the inherent complexities of video data processing, setting new standards for AI's capacity to interpret and interact with multimedia content.
Advancements in Video Understanding
Videos are inherently complex, comprising a sequence of frames that encapsulate both spatial and temporal information. Traditional AI models often face challenges in effectively capturing the dynamic nature of video content, leading to limitations in tasks such as video summarization, captioning, and analysis. Apollo's architecture is specifically designed to overcome these challenges, enabling more accurate and efficient video comprehension.
Multimodal Integration
Beyond video, Apollo's multimodal capabilities allow it to process and integrate various data types, including text and images. This integration is crucial for applications that require a comprehensive understanding of diverse data sources, such as virtual assistants, autonomous vehicles, and content recommendation systems. By effectively combining multiple modalities, Apollo enhances the AI's ability to interpret and respond to complex, real-world scenarios.
Open-Source Accessibility
In line with Meta's commitment to fostering innovation and collaboration, Apollo is released as an open-source model. This approach democratizes access to advanced AI technologies, enabling researchers, developers, and organizations to leverage Apollo's capabilities in their projects. The open-source nature of Apollo encourages community-driven improvements and adaptations, accelerating the pace of innovation in AI applications.
Implications for AI Development
The launch of Apollo signifies a substantial advancement in AI's ability to process and interpret video content. By addressing the complexities inherent in video data, Apollo sets a new benchmark for AI models, paving the way for more intuitive and efficient systems capable of transforming how we interact with and analyze multimedia content.
How does Apollo work?
Apollo is a suite of Large Multimodal Models (LLMs) developed by Meta AI to effectively process and understand video data. Recognizing the inherent complexities of video content—such as the combination of spatial and temporal dimensions—Apollo aims to advance AI's capability to interpret and interact with multimedia information.
Design and Architecture
Apollo's architecture is meticulously crafted to address the unique challenges posed by video data:
Frame Sampling: Instead of relying on uniform frame sampling, Apollo employs a more efficient video sampling technique that ensures better temporal consistency, effectively capturing motion and temporal patterns.
Dual Vision Encoders: Apollo integrates two vision encoders—SigLIP for spatial understanding and InternVideo2 for temporal reasoning—enabling a balanced representation of video data.
Token Resampling: Utilizing a Perceiver Resampler, Apollo efficiently reduces video tokens without losing information, allowing the models to process long videos without excessive computational overhead.
Optimized Training: Apollo employs a three-stage training process where video encoders are initially fine-tuned on video data before integrating with text and image datasets. This staged approach ensures stable and effective learning.
Performance and Benchmarks
Apollo has demonstrated exceptional performance across various benchmarks:
Apollo-1.5B: Surpasses models like Phi-3.5-Vision (4.2B) and LongVA-7B, achieving scores of 60.8 on Video-MME, 63.3 on MLVU, and 57.0 on ApolloBench.
Apollo-3B: Competes with and outperforms many 7B models, with scores of 58.4 on Video-MME, 68.7 on MLVU, and 62.7 on ApolloBench. It also achieves 55.1 on LongVideoBench.
Apollo-7B: Matches and even surpasses models with over 30B parameters, such as Oryx-34B and VILA1.5-40B, with scores of 61.2 on Video-MME, 70.9 on MLVU, and 66.3 on ApolloBench.
Applications and Implications
Apollo's capabilities extend to various applications:
Video Analysis: Apollo's advanced video understanding facilitates more accurate and efficient video analysis, benefiting sectors like entertainment, security, and education.
Multimodal Systems: The models can be integrated into systems requiring the processing of multiple data types, such as virtual assistants and autonomous vehicles.
By addressing the complexities inherent in video data, Apollo sets a new benchmark for AI models, paving the way for more intuitive and efficient systems capable of transforming how we interact with and analyze multimedia content.
The multimodal nature of Apollo allows it to integrate and process information from different sources simultaneously. For instance, in video analysis, Apollo can interpret visual content, understand accompanying audio, and process any embedded textual information, such as subtitles or captions. This holistic approach enhances the model's ability to comprehend complex scenarios and generate more accurate and contextually relevant outputs.
Apollo's design incorporates advanced techniques to handle the intricacies of multimodal data. By leveraging specialized encoders for different data types, the model effectively captures the unique features of each modality. This specialization ensures that Apollo can process and understand diverse inputs, from visual elements in images and videos to linguistic structures in text, facilitating a more nuanced and comprehensive analysis.
The integration of multimodal capabilities in Apollo has significant implications for various fields. In healthcare, for example, Apollo can analyze medical images, interpret patient records, and process spoken descriptions, providing a more complete understanding of patient conditions. In entertainment, the model can analyze video content, understand associated scripts, and process audience feedback, enabling more sophisticated content recommendations and personalized experiences.
Furthermore, Apollo's multimodal capabilities extend to real-time applications. In autonomous vehicles, the model can process visual data from cameras, interpret sensor data, and understand spoken commands, facilitating safer and more efficient navigation. In customer service, Apollo can analyze customer inquiries, understand sentiment, and process visual cues, such as images or videos sent by customers, to provide more accurate and context-aware responses.
The versatility of Apollo's multimodal capabilities also enhances its performance in tasks such as video captioning, question answering, and summarization. By understanding and integrating information from multiple modalities, Apollo can generate more accurate and contextually relevant captions, provide precise answers to complex questions, and create concise summaries that capture the essence of the content.
In summary, Apollo's ability to handle and integrate various data types—including text, images, and video—enables it to perform comprehensive analysis and interaction across multiple modalities. This multimodal capability enhances Apollo's versatility and effectiveness, making it a powerful tool for a wide range of applications.
The architecture of Apollo is optimized to handle the complexities inherent in video data. Unlike traditional models that may rely on uniform frame sampling, Apollo employs a more efficient video sampling technique, ensuring better temporal consistency and effectively capturing motion and sequence of events in videos. This approach allows the model to maintain a consistent temporal flow, which is crucial for understanding the dynamics within video content.
A key feature of Apollo is its dual vision encoders. By combining SigLIP for spatial understanding with InternVideo2 for temporal reasoning, Apollo achieves a balanced representation of video data. This dual-encoder system enables the model to comprehend both the static and dynamic aspects of videos, enhancing its ability to interpret complex visual scenes and sequences.
To efficiently process long videos without excessive computational overhead, Apollo utilizes a Perceiver Resampler. This component reduces video tokens without losing information, allowing the model to handle extended video content effectively. This efficiency is particularly beneficial for applications that require the analysis of lengthy videos, such as surveillance footage or long-form media content.
In terms of performance, Apollo has demonstrated impressive results across various benchmarks. For instance, the Apollo-3B model outperforms many existing 7B models, achieving a score of 55.1 on LongVideoBench. This performance underscores Apollo's capability to comprehend and analyze extended video content with a high degree of accuracy.
The implications of Apollo's advanced video understanding are far-reaching. In sectors such as healthcare, Apollo can analyze medical videos, interpret patient records, and process spoken descriptions, providing a more complete understanding of patient conditions. In entertainment, the model can analyze video content, understand associated scripts, and process audience feedback, enabling more sophisticated content recommendations and personalized experiences. Furthermore, Apollo's capabilities extend to real-time applications, such as autonomous vehicles, where it can process visual data from cameras, interpret sensor data, and understand spoken commands, facilitating safer and more efficient navigation.
In summary, Apollo's design and features position it as a powerful tool for advanced video understanding. Its ability to process and interpret video content with high efficiency and accuracy opens new possibilities for AI applications across various domains.
Technical Specifications
Among its variants, Apollo-3B has demonstrated remarkable performance, particularly in the LongVideoBench benchmark, where it achieved a score of 55.1. This performance surpasses that of many existing 7B models, highlighting Apollo-3B's efficiency and effectiveness in video understanding tasks.
The impressive performance of Apollo-3B can be attributed to several key factors:
Efficient Video Sampling: Apollo employs frame-per-second (fps) sampling during training, which has been shown to be vastly preferable to uniform frame sampling. This method ensures that the model captures the most relevant temporal information, leading to better performance in video understanding tasks.
Advanced Vision Encoders: The model utilizes state-of-the-art vision encoders that are optimized for video representation. These encoders enable Apollo to effectively process and understand the spatial and temporal aspects of video data, contributing to its superior performance.
Scalable Design: Apollo's architecture is designed with scalability in mind, allowing it to efficiently process hour-long videos. This scalability ensures that the model can handle large-scale video data without compromising performance.
In addition to its performance on LongVideoBench, Apollo-3B has achieved notable scores on other benchmarks:
MLVU (Multimodal Language Understanding Benchmark): Apollo-3B scored 68.7, demonstrating its proficiency in understanding and processing multimodal language inputs.
ApolloBench: The model achieved a score of 62.7, further underscoring its capabilities in video understanding tasks.
These achievements position Apollo-3B as a leading model in the field of video understanding, setting new benchmarks for performance and efficiency. Its ability to outperform larger models with fewer parameters underscores the effectiveness of its design and training methodologies.
Apollo-7B, the largest model in Meta AI's Apollo family, has set new benchmarks in video understanding and multimodal processing. Achieving a score of 70.9 on the Multimodal Language Understanding (MLVU) benchmark and 63.3 on Video-MME, Apollo-7B outperforms many existing models, including those with over 30 billion parameters.
The MLVU benchmark evaluates a model's ability to comprehend and process multimodal language inputs, encompassing tasks that require understanding and integrating information from text, images, and videos. Apollo-7B's score of 70.9 signifies a substantial advancement in this area, indicating its proficiency in handling complex multimodal data.
Similarly, the Video-MME benchmark assesses a model's performance in video multimodal understanding, focusing on tasks such as video captioning, summarization, and question answering. With a score of 63.3, Apollo-7B demonstrates its capability to effectively process and interpret video content, setting a new standard for models in this domain.
These achievements underscore Apollo-7B's advanced architecture and training methodologies, which contribute to its superior performance. The model's design incorporates efficient video sampling techniques, dual vision encoders, and optimized training schedules, enabling it to handle large-scale video data and complex multimodal tasks effectively.
The implications of Apollo-7B's performance are significant across various applications. In healthcare, for instance, the model's ability to analyze medical videos and interpret patient records can enhance diagnostic accuracy and patient care. In entertainment, Apollo-7B can process video content, understand associated scripts, and analyze audience feedback, facilitating more sophisticated content recommendations and personalized experiences.
Furthermore, Apollo-7B's capabilities extend to real-time applications, such as autonomous vehicles, where it can process visual data from cameras, interpret sensor data, and understand spoken commands, contributing to safer and more efficient navigation. In customer service, the model can analyze customer inquiries, understand sentiment, and process visual cues, such as images or videos sent by customers, to provide more accurate and context-aware responses.
In summary, Apollo-7B's performance on the MLVU and Video-MME benchmarks highlights its advanced capabilities in multimodal understanding and video processing. Its ability to outperform larger models with fewer parameters underscores the effectiveness of its design and training strategies, setting a new standard for AI models in these domains.
Apollo, developed by Meta AI, represents a significant advancement in the field of Large Multimodal Models (LLMs), particularly in the realm of video understanding. Designed to process and comprehend hour-long videos, Apollo addresses the inherent complexities of video data, which combines both spatial and temporal dimensions. Traditional models often struggle with the vast amount of information present in lengthy videos, leading to challenges in capturing nuanced patterns and sequences. Apollo's architecture is specifically optimized to handle these challenges, enabling it to efficiently process extensive video content.
A key aspect of Apollo's design is its training methodology, which leverages extensive datasets to capture a wide array of spatial and temporal patterns. By training on diverse and comprehensive video data, Apollo learns to recognize and interpret complex sequences and interactions within videos. This extensive training allows the model to develop a deep understanding of video content, facilitating tasks such as video summarization, captioning, and analysis.
The model's architecture incorporates advanced vision encoders that are tailored for video representation. These encoders are designed to process both spatial and temporal information effectively, ensuring that Apollo can capture the dynamic nature of video content. The integration of these specialized encoders enables Apollo to maintain a consistent temporal flow, which is crucial for understanding the dynamics within video content.
To further enhance its efficiency, Apollo employs a Perceiver Resampler. This component reduces video tokens without losing information, allowing the model to handle extended video content effectively. This efficiency is particularly beneficial for applications that require the analysis of lengthy videos, such as surveillance footage or long-form media content.
In terms of performance, Apollo has demonstrated impressive results across various benchmarks. For instance, the Apollo-3B model outperforms many existing 7B models, achieving a score of 55.1 on LongVideoBench. This performance underscores Apollo's capability to comprehend and analyze extended video content with a high degree of accuracy.
The implications of Apollo's advanced video understanding are far-reaching. In sectors such as healthcare, Apollo can analyze medical videos, interpret patient records, and process spoken descriptions, providing a more complete understanding of patient conditions. In entertainment, the model can analyze video content, understand associated scripts, and process audience feedback, enabling more sophisticated content recommendations and personalized experiences. Furthermore, Apollo's capabilities extend to real-time applications, such as autonomous vehicles, where it can process visual data from cameras, interpret sensor data, and understand spoken commands, facilitating safer and more efficient navigation.
In summary, Apollo's design and features position it as a powerful tool for advanced video understanding. Its ability to process and interpret video content with high efficiency and accuracy opens new possibilities for AI applications across various domains.
Applications and Implications
Apollo, developed by Meta AI, represents a significant advancement in video understanding and multimodal processing, offering enhanced capabilities that benefit various sectors, including entertainment, security, and education. Its ability to process and comprehend hour-long videos with high efficiency and accuracy has opened new possibilities for applications across these industries.
In the entertainment industry, Apollo's advanced video analysis capabilities enable more accurate and efficient content analysis. By processing extensive video data, Apollo can assist in tasks such as content summarization, sentiment analysis, and audience engagement assessment. This facilitates the creation of personalized content recommendations and enhances user experiences across platforms. For instance, Apollo's ability to analyze viewer preferences and interactions can inform content creation strategies, leading to more engaging and relevant media offerings.
In the security sector, Apollo's proficiency in video analysis enhances surveillance systems by enabling real-time monitoring and threat detection. Its capability to process and interpret video feeds from multiple sources allows for the identification of unusual activities, potential security breaches, and the tracking of individuals across various locations. This contributes to more effective security measures and rapid response to incidents. Additionally, Apollo's ability to analyze historical video data aids in investigations, providing valuable insights that support law enforcement and security agencies in their operations.
In the education sector, Apollo's capabilities facilitate the analysis of educational videos, enabling the extraction of key information and insights. This supports the development of interactive learning materials, personalized educational content, and the assessment of teaching effectiveness. By understanding and processing educational videos, Apollo can assist in creating adaptive learning environments that cater to individual student needs, enhancing the overall learning experience.
Overall, Apollo's enhanced video analysis capabilities offer significant benefits across various sectors, enabling more accurate, efficient, and insightful processing of video content. Its ability to handle complex video data with high efficiency and accuracy positions it as a valuable tool for advancing applications in entertainment, security, education, and beyond.
Apollo, developed by Meta AI, represents a significant advancement in Large Multimodal Models (LLMs), particularly in the realm of video understanding. Its design and capabilities make it highly suitable for integration into systems that require the processing of multiple data types, such as virtual assistants and autonomous vehicles.
In the context of virtual assistants, Apollo's multimodal processing abilities enable it to handle diverse inputs, including text, images, and video. This versatility allows virtual assistants powered by Apollo to engage in more natural and context-aware interactions with users. For instance, a user could provide a voice command accompanied by a photo, and the assistant could process both the visual and auditory information to deliver a comprehensive response. This integration enhances the assistant's ability to understand and respond to complex queries, improving user satisfaction and engagement.
Moreover, Apollo's proficiency in understanding and processing video content allows virtual assistants to offer more dynamic and interactive experiences. For example, when integrated into smart glasses, Apollo can analyze the user's environment in real-time, providing contextual information and assistance. This capability is particularly beneficial in applications such as navigation, where the assistant can process live video feeds to offer turn-by-turn directions or identify points of interest in the user's vicinity. Additionally, Apollo's ability to process and understand video content enables virtual assistants to offer more dynamic and interactive experiences.
In the realm of autonomous vehicles, Apollo's multimodal capabilities are equally transformative. Autonomous driving systems require the integration of various data types, including visual inputs from cameras, sensor data from LiDAR and radar, and contextual information such as traffic rules and user preferences. Apollo's design allows it to process and synthesize these diverse data streams, facilitating more accurate and efficient decision-making in autonomous vehicles.
For instance, Apollo can analyze video feeds from multiple cameras to detect and recognize pedestrians, other vehicles, and traffic signals, while simultaneously processing sensor data to assess the vehicle's surroundings. This comprehensive understanding enables the vehicle to navigate complex environments safely and effectively. Furthermore, Apollo's ability to understand and process video content enhances the vehicle's situational awareness, allowing it to respond appropriately to dynamic changes in the environment.
The integration of Apollo into autonomous vehicles also supports advanced features such as predictive maintenance and personalized user experiences. By analyzing video and sensor data, Apollo can detect anomalies or potential issues in the vehicle's systems, enabling proactive maintenance and reducing the likelihood of unexpected failures. Additionally, Apollo can process user preferences and behaviors to customize the driving experience, adjusting settings such as seat position, climate control, and infotainment options to suit individual preferences.
Comparison with Competitors
Meta AI's Apollo, OpenAI's Sora, and Google's Veo 2 represent the forefront of AI-driven video understanding and generation technologies, each offering unique capabilities and positioning within the market.
Apollo distinguishes itself with its advanced video comprehension, enabling the processing and interpretation of hour-long videos. This capability is particularly beneficial for applications requiring in-depth analysis of extended video content, such as surveillance, long-form media analysis, and comprehensive educational materials. Apollo's design allows it to capture both spatial and temporal patterns within videos, facilitating tasks like video summarization, captioning, and detailed content analysis. This positions Apollo as a powerful tool for industries that rely on extensive video data processing.
In contrast, OpenAI's Sora focuses on generating high-quality videos from textual descriptions, with a maximum output resolution of 1080p. While Sora excels in creating realistic and contextually relevant video content based on user prompts, its capabilities are currently limited to shorter video segments. This makes Sora particularly useful for applications in content creation, marketing, and entertainment, where quick generation of video snippets is advantageous. However, the shorter video length and lower resolution compared to Veo 2 may limit its applicability in scenarios requiring longer or higher-quality video outputs.
Google's Veo 2, on the other hand, has introduced significant advancements in AI-driven video generation. It offers the ability to produce videos in 4K resolution, surpassing both Apollo and Sora in visual quality. Veo 2 also supports longer video durations, extending to several minutes, which enhances its utility in applications such as cinematic content creation, detailed tutorials, and comprehensive marketing materials. Additionally, Veo 2 responds to specific filmmaking instructions, including different types of lenses and camera effects, providing users with greater creative control over the generated content. This positions Veo 2 as a formidable competitor in the AI video generation market, offering high-resolution outputs and extended video lengths that cater to professional content creators and industries requiring high-quality video production.
In summary, while Apollo, Sora, and Veo 2 each offer distinct advantages, their positioning in the market reflects their specialized capabilities. Apollo's strength lies in its advanced video understanding and processing of long-form content, making it ideal for applications requiring in-depth analysis of extensive video data. Sora excels in generating realistic short videos from textual prompts, suitable for quick content creation needs. Veo 2 leads in high-resolution video generation with extended durations, catering to professional content creators seeking high-quality outputs. The choice between these models depends on the specific requirements of the application, including factors such as video length, resolution, and the need for video generation versus analysis.
Meta AI's Movie Gen model has demonstrated superior performance in human evaluations compared to its competitors, including OpenAI's Sora and Google's Veo. In assessments focusing on video quality, motion realism, naturalness, and consistency, Movie Gen has been noted to surpass rival models.
Evaluations of Movie Gen have been conducted using human ratings on a Likert scale, providing a comprehensive assessment of the model's ability to handle complex temporal dynamics. These evaluations consider various aspects, such as alignment with the input prompt, quality and consistency, and the realism and aesthetics of the generated videos.
In terms of video quality, Movie Gen has been recognized for producing realistic, personalized HD videos of up to 16 seconds at 16 frames per second, accompanied by 48kHz audio. This capability enables the creation of high-quality video content suitable for various applications, including personalized video creation, sophisticated video editing, and high-quality audio generation.
The model's performance in motion realism and alignment with input text has been evaluated through human assessments, demonstrating state-of-the-art performance in text-to-motion generation. This indicates that Movie Gen effectively captures and reproduces motion dynamics described in textual prompts, contributing to the naturalness and consistency of the generated videos.
Overall, Movie Gen's performance metrics highlight its advanced capabilities in video generation, particularly in producing high-quality, realistic, and consistent videos that align well with user prompts. These attributes position Movie Gen as a leading model in the AI video generation market, offering unique advantages in video understanding and multimodal integration.
Future Prospects
Meta AI's Apollo model represents a significant advancement in video understanding and multimodal integration, with ongoing developments aimed at expanding its capabilities and applications across various industries. Since its introduction, Meta has been actively refining Apollo to enhance its performance, scalability, and versatility.
One of the key areas of focus has been improving Apollo's efficiency in processing longer video content. The model is designed to handle videos up to an hour long, enabling comprehensive analysis of extended video data. This capability is particularly beneficial for applications in sectors such as surveillance, entertainment, and education, where long-form video analysis is essential. By enhancing Apollo's ability to process lengthy videos, Meta aims to provide more accurate and detailed insights, thereby increasing the model's utility across various domains.
In addition to extending video length capabilities, Meta has been working on optimizing Apollo's performance across different model sizes. The Apollo family includes models with 1.5B, 3B, and 7B parameters, offering flexibility to accommodate various computational constraints and real-world needs. This scalability ensures that Apollo can be effectively utilized in diverse environments, from resource-constrained devices to high-performance computing systems. By providing options that balance performance and resource requirements, Meta enables a broader range of applications for Apollo.
Meta has also been enhancing Apollo's multimodal capabilities, enabling it to process and understand various data types, including text, images, and video. This integration allows for more comprehensive analysis and interaction, facilitating tasks such as video summarization, captioning, and analysis. By improving Apollo's ability to handle multiple data modalities, Meta aims to support a wide array of applications, from virtual assistants to autonomous vehicles, thereby broadening the model's applicability across different industries.
Furthermore, Meta has been focusing on refining Apollo's temporal reasoning abilities. The model's design incorporates advanced temporal reasoning techniques, enabling it to understand and interpret the sequence and timing of events within videos. This enhancement is crucial for applications that require a deep understanding of temporal dynamics, such as video-based question answering and event detection. By improving temporal reasoning, Meta aims to provide more accurate and context-aware analyses, thereby increasing the model's effectiveness in complex video understanding tasks.
To support these advancements, Meta has been developing and implementing new training methodologies and data loading techniques. For instance, the introduction of Scalable and Performant Data Loading (SPDL) has significantly improved data throughput, leading to faster training times for Apollo. SPDL boosts data throughput by 3-5 times compared to traditional process-based data loaders, translating to up to 30% faster training times. This improvement accelerates the development cycle, allowing for more rapid iterations and enhancements to the model.
In terms of real-world applications, Meta has been integrating Apollo into various products and services. For example, the integration of Apollo into smart glasses has enabled real-time AI video processing, enhancing the user experience by providing immediate insights and interactions based on the visual context. This integration demonstrates Apollo's potential in wearable technology, offering users enhanced functionalities such as augmented reality experiences and context-aware assistance.
Looking ahead, Meta plans to continue refining Apollo, focusing on expanding its capabilities and applications across various industries. Ongoing developments aim to enhance the model's performance, scalability, and versatility, ensuring that Apollo remains at the forefront of AI-driven video understanding and multimodal integration. By addressing the evolving needs of different sectors, Meta seeks to provide innovative solutions that leverage Apollo's advanced capabilities, thereby driving progress in AI technology and its practical applications.
Meta AI's Apollo model represents a significant advancement in artificial intelligence, particularly in the realm of video understanding and multimodal integration. By effectively processing and interpreting hour-long videos, Apollo addresses the inherent complexities of video data, which combines spatial and temporal dimensions that demand substantial computational resources.
The potential impact of Apollo is vast, with the model poised to transform various industries by enabling more intuitive and efficient AI systems. In sectors such as entertainment, Apollo's advanced video comprehension could revolutionize content creation and analysis. For instance, filmmakers and content creators could leverage Apollo to automate the editing process, generate detailed video summaries, and enhance the personalization of content for diverse audiences. This would not only streamline production workflows but also open new avenues for creative expression and audience engagement.
In the field of security, Apollo's ability to analyze extensive video footage could significantly improve surveillance systems. The model's proficiency in detecting and interpreting complex events within long-duration videos would enable more effective monitoring and response strategies. For example, Apollo could assist in identifying unusual activities or potential threats in real-time, thereby enhancing public safety and security operations.
Education is another domain that stands to benefit from Apollo's capabilities. The model's advanced video understanding could facilitate the creation of interactive and immersive learning experiences. Educators could utilize Apollo to develop educational videos that adapt to individual learning styles and preferences, providing personalized instruction that enhances student engagement and comprehension.
Moreover, Apollo's integration into multimodal systems could lead to more sophisticated virtual assistants and autonomous vehicles. By processing and understanding various data types, including text, images, and video, Apollo could enable these systems to interact more naturally and effectively with users. For instance, a virtual assistant powered by Apollo could interpret visual cues from its environment, allowing for more context-aware and responsive interactions.
The advancements in AI models like Apollo also raise important considerations regarding ethical implications and responsible deployment. As AI systems become more capable, it is crucial to address concerns related to privacy, security, and the potential for misuse. Ensuring that models like Apollo are developed and implemented with robust ethical guidelines will be essential to maximize their benefits while mitigating risks.
In summary, the development of Apollo signifies a substantial leap forward in AI's ability to understand and process video content. Its potential to transform industries such as entertainment, security, education, and autonomous systems underscores the importance of ongoing research and development in this field. As Meta AI continues to refine Apollo, the model's integration into various applications promises to enhance the way we interact with and analyze video content, leading to more intuitive and efficient AI systems across diverse sectors.
Conclusion
Meta AI's Apollo model represents a significant advancement in artificial intelligence, particularly in the realm of video understanding and multimodal integration. By effectively processing and interpreting hour-long videos, Apollo addresses the inherent complexities of video data, which combines spatial and temporal dimensions that demand substantial computational resources.
The potential impact of Apollo is vast, with the model poised to transform various industries by enabling more intuitive and efficient AI systems. In sectors such as entertainment, Apollo's advanced video comprehension could revolutionize content creation and analysis. For instance, filmmakers and content creators could leverage Apollo to automate the editing process, generate detailed video summaries, and enhance the personalization of content for diverse audiences. This would not only streamline production workflows but also open new avenues for creative expression and audience engagement.
In the field of security, Apollo's ability to analyze extensive video footage could significantly improve surveillance systems. The model's proficiency in detecting and interpreting complex events within long-duration videos would enable more effective monitoring and response strategies. For example, Apollo could assist in identifying unusual activities or potential threats in real-time, thereby enhancing public safety and security operations.
Education is another domain that stands to benefit from Apollo's capabilities. The model's advanced video understanding could facilitate the creation of interactive and immersive learning experiences. Educators could utilize Apollo to develop educational videos that adapt to individual learning styles and preferences, providing personalized instruction that enhances student engagement and comprehension.
Moreover, Apollo's integration into multimodal systems could lead to more sophisticated virtual assistants and autonomous vehicles. By processing and understanding various data types, including text, images, and video, Apollo could enable these systems to interact more naturally and effectively with users. For instance, a virtual assistant powered by Apollo could interpret visual cues from its environment, allowing for more context-aware and responsive interactions.
The advancements in AI models like Apollo also raise important considerations regarding ethical implications and responsible deployment. As AI systems become more capable, it is crucial to address concerns related to privacy, security, and the potential for misuse. Ensuring that models like Apollo are developed and implemented with robust ethical guidelines will be essential to maximize their benefits while mitigating risks.
In summary, the development of Apollo signifies a substantial leap forward in AI's ability to understand and process video content. Its potential to transform industries such as entertainment, security, education, and autonomous systems underscores the importance of ongoing research and development in this field. As Meta AI continues to refine Apollo, the model's integration into various applications promises to enhance the way we interact with and analyze video content, leading to more intuitive and efficient AI systems across diverse sectors.
Here are some reliable sources and references for learning more about Meta AI’s Apollo model and its impact on video understanding and multimodal applications:
Meta AI Blog – Official blog posts from Meta detailing Apollo and other advancements in AI technology.
Stanford AI Research – Collaborative efforts between Meta AI and Stanford University on video AI and large multimodal models.
MarkTechPost – Articles and updates on AI advancements, including Meta's Apollo model and its use in various industries.
MIT Technology Review – Articles and deep dives on cutting-edge AI technologies, including video comprehension models like Apollo.
Nature AI – Research papers and insights into multimodal models and video understanding, covering major breakthroughs in the AI field.
arXiv.org – Open-access research papers on Apollo, video AI models, and multimodal systems. Useful for finding in-depth academic research.
VentureBeat AI Section – News, analysis, and opinion on artificial intelligence and its applications, including video models.
VentureBeat AI
TechCrunch AI Section – Covers AI-related launches, including Meta's innovations in video models and other multimodal technologies.
TechCrunch AI
OpenAI Blog – While not directly related to Apollo, OpenAI's blog provides valuable context on large multimodal models and the general direction of AI research.
YouTube Video: New Video AI by META & Stanford Univ: APOLLO (7B) – A visual and in-depth look at Apollo's capabilities and the research behind it.
These references will provide a blend of theoretical background, industry news, and academic research related to Apollo and the broader field of multimodal AI systems.
Press contact
Timon Harz
oneboardhq@outlook.com
Other posts
Company
About
Blog
Careers
Press
Legal
Privacy
Terms
Security