Timon Harz
December 16, 2024
Introducing SRDF: A Self-Refining Data Flywheel for High-Quality Vision-and-Language Navigation Datasets in AI Research
Discover the power of SRDF in AI research and how it addresses common challenges in data management. Uncover how this self-refining framework enhances the quality of datasets and drives breakthrough advancements in vision-and-language navigation tasks.
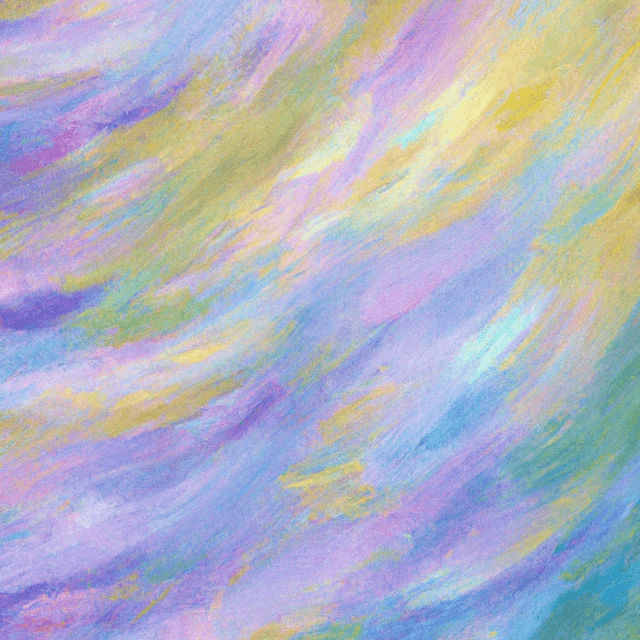
Vision-and-Language Navigation (VLN) integrates visual perception with natural language processing to enable agents to navigate 3D environments by interpreting human-like instructions. This technology is crucial for applications in robotics, augmented reality, and smart assistants, where the interaction with physical spaces is guided by linguistic commands. VLN aims to improve how agents understand and execute complex navigational tasks, making it a key area of research for advancing intelligent systems.
A major challenge in VLN is the scarcity of high-quality datasets that pair accurate navigation paths with natural language instructions. The process of manually annotating these datasets is resource-intensive, requiring significant time and expert knowledge. Furthermore, these annotations often lack the depth and complexity necessary for training models that generalize well across diverse real-world environments, limiting the practical use of VLN systems.
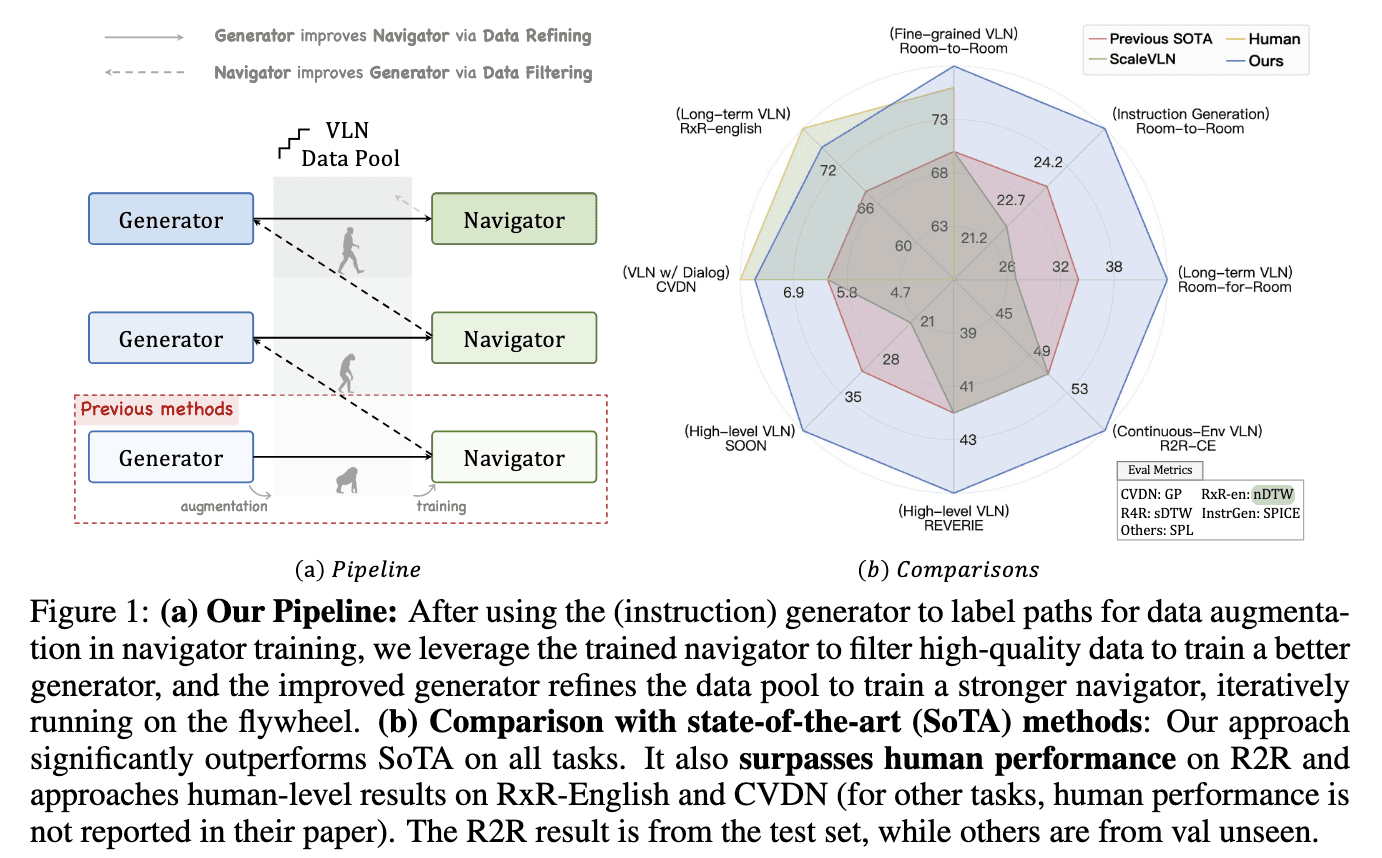
Existing solutions to Vision-and-Language Navigation (VLN) often rely on synthetic data generation and environmental augmentation techniques. Synthetic data is produced through trajectory-to-instruction models, while simulators create diverse environments. However, these methods often fall short in terms of quality, resulting in misaligned data between linguistic instructions and navigation trajectories. This misalignment leads to suboptimal agent performance. Additionally, existing evaluation metrics often fail to effectively assess the semantic and directional alignment of instructions with their corresponding trajectories, complicating quality control processes.
To address these challenges, researchers from Shanghai AI Laboratory, UNC Chapel Hill, Adobe Research, and Nanjing University introduced the Self-Refining Data Flywheel (SRDF). SRDF is an innovative system that fosters mutual collaboration between an instruction generator and a navigator, enabling iterative improvements in both the dataset and models. This fully automated approach eliminates the need for human-in-the-loop annotation. Starting with a small, high-quality human-annotated dataset, SRDF generates synthetic instructions and uses them to train a base navigator. The navigator then evaluates the fidelity of these instructions, filtering out low-quality data and refining the instruction generator over successive iterations. This iterative process ensures ongoing improvements in both data quality and the performance of the models.
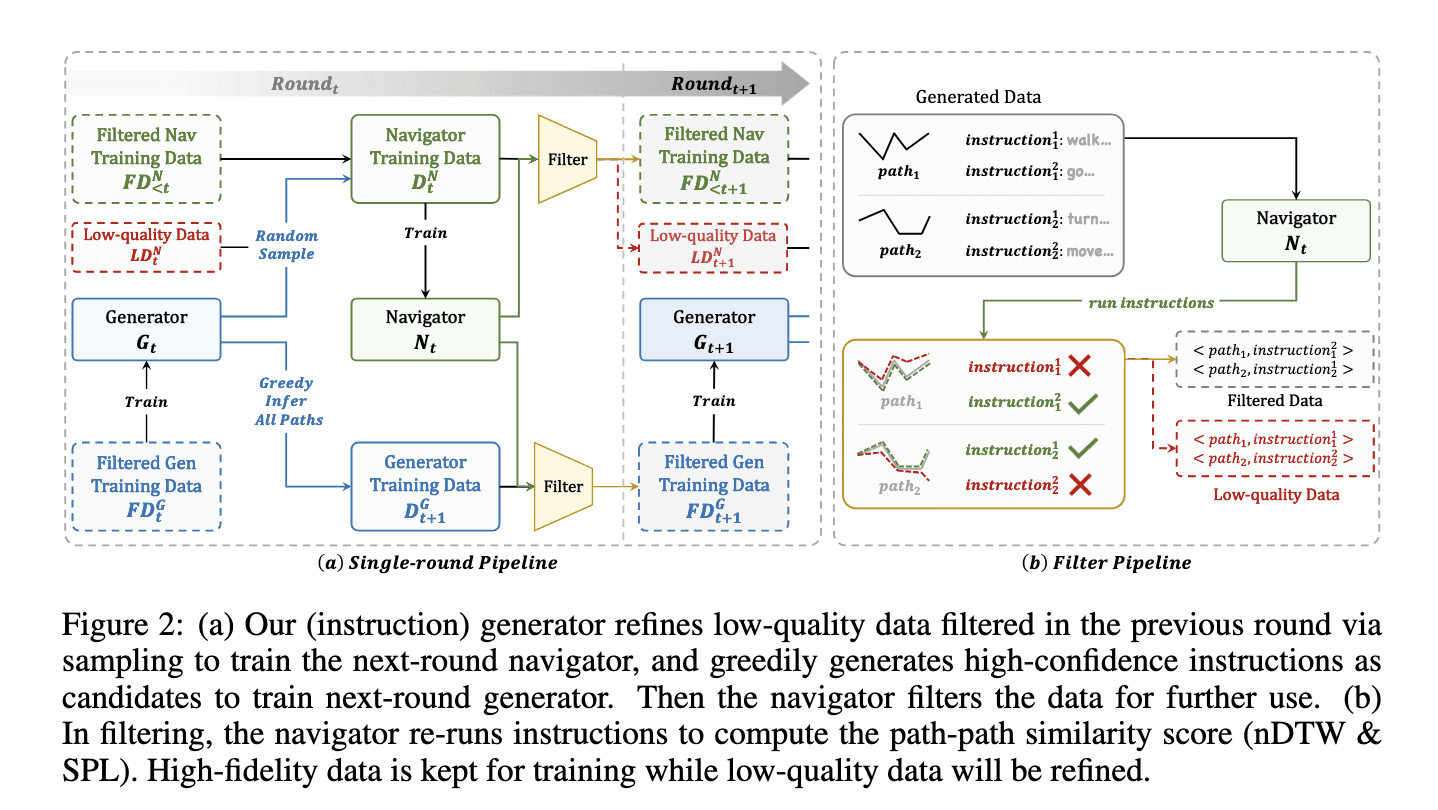
The SRDF system consists of two integral components: an instruction generator and a navigator. The instruction generator leverages advanced multimodal language models to create synthetic navigation instructions based on trajectories. The navigator evaluates these instructions by measuring its ability to accurately follow the generated paths. To ensure high-quality data, the system applies stringent fidelity metrics such as Success weighted by Path Length (SPL) and normalized Dynamic Time Warping (nDTW). Data that does not meet the quality standards is either regenerated or excluded, ensuring that only highly reliable and well-aligned data is used for training. Through three iterative cycles, the SRDF system refines the dataset, ultimately producing 20 million high-quality instruction-trajectory pairs across 860 diverse environments.
The SRDF system has shown remarkable performance improvements across various benchmarks and metrics. On the Room-to-Room (R2R) dataset, the SPL metric for the navigator increased from 70% to an impressive 78%, surpassing the human benchmark of 76%. This achievement marks the first time a VLN agent has outperformed human-level navigation accuracy. Additionally, the instruction generator achieved significant gains, with SPICE scores improving from 23.5 to 26.2, outperforming all previous Vision-and-Language Navigation instruction generation methods. The SRDF-generated data also enabled superior generalization across downstream tasks, including long-term navigation (R4R) and dialogue-based navigation (CVDN), setting new state-of-the-art performance records across all tested datasets.
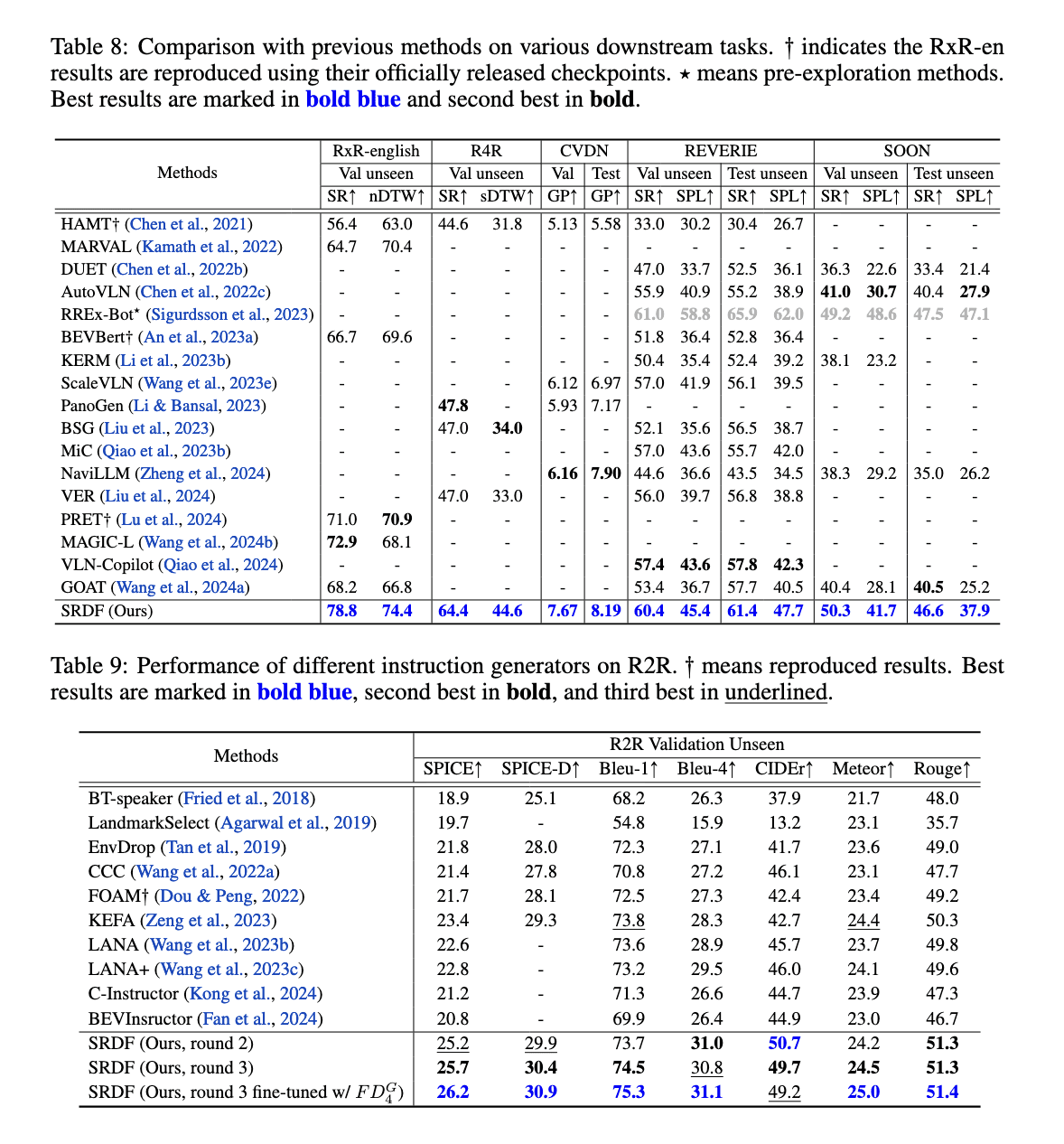
The SRDF system demonstrated exceptional performance in long-horizon navigation, achieving a 16.6% improvement in Success Rate on the R4R dataset. In the CVDN dataset, the system significantly enhanced the Goal Progress metric, outperforming all prior models. Moreover, SRDF’s scalability was evident, with the instruction generator consistently improving as larger datasets and more diverse environments were incorporated. This ensured robust performance across various tasks and benchmarks. The researchers also reported an increase in instruction diversity and richness, incorporating over 10,000 unique words into the SRDF-generated dataset, overcoming the vocabulary limitations of previous datasets.
By automating dataset refinement, the SRDF approach tackles the long-standing issue of data scarcity in Vision-and-Language Navigation. The ongoing iterative collaboration between the navigator and the instruction generator ensures the continuous enhancement of both components, resulting in highly aligned and high-quality datasets. This groundbreaking method has set a new standard in VLN research, highlighting the importance of data quality and alignment in the development of embodied AI systems. With its ability to surpass human performance and generalize across a wide range of tasks, SRDF is positioned to accelerate advancements in intelligent navigation systems.
Creating high-quality vision-and-language navigation (VLN) datasets is a complex and ongoing challenge in AI research, requiring the integration of diverse data sources and methodologies to achieve realistic and effective navigation. VLN tasks typically involve an agent navigating an environment by interpreting natural language instructions, which is no simple task. These environments can vary significantly, from simple indoor settings to complex outdoor scenes, making the task of collecting accurate, diverse, and sufficiently large datasets particularly difficult.
One of the primary obstacles is the challenge of generating high-quality natural language instructions that are both grounded in the visual environment and comprehensible to the agent. Early approaches often struggled with poorly structured instructions that were either too vague or difficult to follow. For instance, instructions like "go to the kitchen" may be too general to offer clear guidance in a complex environment with many potential paths, making it difficult for an agent to reliably navigate. More recent methods have aimed to refine these instructions through data augmentation, including the use of synthetic data and environments that simulate real-world scenarios more effectively.
Additionally, many datasets have been limited in scale and scope. For instance, outdoor datasets, which often feature photo-realistic environments like those used in StreetLearn and TOUCHDOWN, require careful attention to detail to maintain realism and ensure that agents can generalize across environments. However, scaling these datasets while preserving quality is a daunting task, especially when synthetic environments are introduced. To overcome these limitations, researchers have turned to strategies such as using imitation learning to train agents on much larger datasets, sometimes involving millions of instructions.
Another challenge lies in the diversity of the navigation tasks themselves. Some navigation tasks are simple, with coarse-grained instructions, while others involve highly detailed and specific instructions that require fine-grained actions. Datasets like ALFRED have explored household tasks with interactive components, where agents must navigate and manipulate objects based on instructions, further complicating the creation of robust datasets.
To summarize, developing high-quality VLN datasets is hindered by the need for both high-quality, natural language instruction data and a diversity of environments. These datasets must balance realism, scale, and the richness of instruction, which often requires innovative approaches such as synthetic data generation and large-scale annotation efforts. Researchers continue to explore ways to create datasets that are not only extensive but also nuanced enough to train AI agents capable of understanding and navigating complex real-world scenarios.
The quality of data is foundational when it comes to training AI models for complex tasks, particularly those involving both vision and language understanding. In these domains, high-quality data directly influences how well the model can understand, interpret, and make predictions about the real world.
When training AI models for vision-and-language tasks, the importance of using accurate and representative data cannot be overstated. First, accuracy is a critical factor—poor-quality data can lead to inaccurate predictions and poor model performance. The quality of the annotations, such as labels and metadata, determines how well the AI can associate visual elements with corresponding language patterns. Without high-quality labels, AI models risk learning from ambiguous or erroneous data, which can lead to misinterpretation of visual cues or linguistic elements.
Moreover, data generalization is another key reason for emphasizing high-quality data. AI models need to be able to apply what they've learned from a specific dataset to new, unseen data. If the training data is sparse, biased, or overly specific, the model may fail to generalize, limiting its usefulness in real-world applications. High-quality, diverse datasets allow models to handle a wider range of inputs, ensuring that they perform well across varied situations.
Another critical issue is bias in the data. AI systems trained on biased datasets may perpetuate these biases in their predictions, which can have real-world consequences. For example, if the data does not adequately represent diverse groups, the model might make inaccurate predictions for underrepresented populations. Mitigating bias through well-curated, inclusive data is essential for fairness and robustness.
Additionally, models trained on high-quality data tend to be more robust and capable of handling unexpected variations in input. This is especially important in complex tasks like vision-and-language navigation, where the environment or language used may vary widely. A dataset with sufficient variety ensures that the model can adapt to new, unseen circumstances.
Ultimately, high-quality data is not just about the quantity of information but also the annotation process. Expert annotators, ideally with subject matter expertise, are crucial in curating and labeling data. Human judgment ensures that the data is correctly interpreted, which is especially important in complex multimodal tasks like vision and language understanding. This human-AI symbiosis in the curation process helps improve the overall performance and reliability of the model, setting a solid foundation for more accurate and fair AI systems.
In short, the quality of data used for training AI models is one of the most important factors determining the model’s success in vision-and-language tasks. Investing in diverse, accurate, and expertly annotated datasets is critical for developing robust AI systems that can be trusted to perform effectively and ethically in real-world applications.
What is SRDF?
The Self-Refining Data Flywheel (SRDF) is an innovative concept designed to address the challenge of generating high-quality, large-scale datasets in AI research, particularly in vision-and-language navigation tasks. The core idea behind SRDF is to continuously improve the quality of training data by using a feedback loop that involves two primary models: an instruction generator and a navigation model. This process begins with an initial data pool created by the instruction generator, which is then used to train a base navigator model. Once trained, the navigator model is applied to refine the dataset by filtering out poor-quality or irrelevant data, allowing the instruction generator to produce even more accurate and detailed instructions for the next iteration of the navigator. This iterative process leads to the creation of a self-improving dataset without the need for human-in-the-loop annotations.
SRDF is grounded in the concept of a "data flywheel," a self-reinforcing mechanism where each round of refinement produces better quality data, which in turn enhances model performance. For instance, in the context of vision-and-language navigation (VLN), this approach results in higher-quality instruction-trajectory pairs that allow the model to better understand and perform navigation tasks based on natural language instructions. The continuous refinement through SRDF not only enhances the effectiveness of the dataset but also significantly boosts the performance of the models involved.
In practice, the SRDF methodology has shown remarkable results. For example, in experiments, the performance of the navigation model improved from 70% to 78% on standard benchmark tests, surpassing human-level performance, while the instruction generator also saw improvements, outperforming previous models tailored for VLN. The key advantage of SRDF is its ability to scale and adapt to a diverse range of environments and tasks, making it a powerful tool for large-scale AI projects where high-quality data is crucial for training robust models. This self-refining approach is particularly valuable in scenarios where human annotation is costly or infeasible, making SRDF an efficient alternative for continuously improving AI models.
The SRDF (Self-Refining Data Flywheel) enhances the iterative improvement of datasets through automation and continuous feedback loops, revolutionizing how AI systems process and refine data over time. The core strength of SRDF lies in its ability to automate the process of dataset enhancement. By using advanced data collection and analysis techniques, SRDF allows AI models to monitor their own outputs, identify patterns, and dynamically improve over time without requiring manual intervention. This ongoing refinement is especially critical in vision-and-language navigation tasks, where dataset quality directly impacts model performance.
The feedback loops play a crucial role in SRDF’s success. These loops allow the system to assess its outputs, compare them to the expected results, and adjust accordingly. This iterative process is akin to a feedback-driven cycle where data insights lead to improvements, which in turn generate new data for further analysis. With every cycle, SRDF helps the dataset evolve, ensuring that the data becomes more accurate, diverse, and robust. In AI, this is vital as data quality dictates how well a model can learn and adapt to complex real-world environments.
In terms of automation, SRDF supports continuous model training and retraining. This process helps in dealing with shifts in data distributions, ensuring that the model adapts to changes in real-time. Automated data ingestion systems enable the quick integration of new data into the model training pipeline, reducing delays and ensuring that models are always updated with the most relevant information. Furthermore, SRDF’s automation ensures that the feedback loops are optimized for efficiency. By continuously tracking the performance of AI models through metrics and real-time data, it can pinpoint areas of improvement without manual oversight, leading to faster refinement cycles.
In summary, SRDF’s integration of feedback loops and automation offers a scalable solution for the iterative improvement of datasets, making it a powerful tool for enhancing vision-and-language navigation datasets and improving AI model accuracy over time. This process of continuous feedback and data optimization drives the creation of high-quality, reliable datasets that are crucial for AI research and application.
The Role of Data Flywheels in AI Research
The concept of data flywheels in machine learning (ML) revolves around a self-sustaining loop that improves both the datasets and the models over time, leading to exponential performance gains. The central idea is that as a model is trained and used in real-world applications, it generates more data that can be used to refine the model. This, in turn, helps the model make better predictions, which prompts further data generation, creating a continuous feedback loop of improvement.
A flywheel is typically a mechanical device that stores energy, providing rotational inertia to keep a system moving smoothly even when the primary input is reduced. In the context of ML, this idea is applied to data and algorithms: data generated by user interactions or system processes is used to refine machine learning models. The key is that each cycle of data collection, model training, and deployment improves the overall system. For example, Google Translate famously used this concept by collecting user feedback on translations, which in turn was used to refine the translation model. This self-reinforcing cycle made the translation engine more effective over time, which encouraged more user interaction, leading to even better data and further refinements.
The data flywheel effect relies on improving both the quality and quantity of the data used to train the model. As new data is collected, it must add value rather than merely replicating existing information. In ML, this means focusing on data that covers weakly represented areas or introduces novel perspectives, which prevents overfitting to repetitive data. For example, in the case of training a model to recognize cats, the model doesn't benefit from seeing the same images repeatedly but gains new insights from different images under varying conditions. This diversity enriches the dataset, pushing the model towards better generalization.
In practice, data flywheels can be used in a variety of ML applications, from improving recommendation systems to enhancing computer vision tasks. Each interaction or new dataset improves the system's ability to make accurate predictions, increasing the overall value of the data generated, which in turn enhances future performance. This is especially important in AI research and applications like vision-and-language navigation, where high-quality datasets are essential for creating models that can understand and navigate the world in a human-like way.
In real-world applications, the benefits of a self-refining data flywheel (SRDF) for AI-powered systems are substantial, particularly in object detection and navigation technologies.
In object detection, SRDFs enable more accurate and scalable systems by improving how models learn and evolve. These systems rely heavily on vast datasets that must be continuously updated and refined. For example, in autonomous vehicles, SRDFs can help by creating and optimizing models that detect pedestrians, road signs, and other vehicles. As data accumulates through real-world driving, these systems can refine their understanding of objects in varying environments, ensuring that autonomous cars can respond to unexpected or rare scenarios. This approach is key to ensuring high precision and adaptability in dynamic, real-time environments.
In navigation systems, particularly those using vision-based inputs, SRDFs can dramatically enhance performance. For instance, robots or drones navigating through complex environments (e.g., warehouses or forests) benefit from the SRDF by continuously learning from their interactions with objects and obstacles. The flywheel effect ensures that as more navigational data is collected, the system’s models evolve, providing increasingly accurate path-planning and obstacle-avoidance capabilities. This is vital in industries like logistics, where efficiency and safety are paramount, and in autonomous vehicles, where split-second decisions can be critical.
Additionally, object detection and navigation systems both rely on feature-rich datasets that can adapt to specific tasks. The SRDF's self-refining nature ensures that such systems evolve in response to new data, enabling a continual enhancement in their ability to detect and navigate through the physical world. Over time, this improves the model's generalization across various environments, reducing the need for manually curated training sets.
How SRDF Works
The Self-Refining Data Flywheel (SRDF) leverages a robust, automated data annotation system that incorporates various cutting-edge techniques to enhance dataset quality and accelerate AI model performance. This process is centered around continuous data refinement, where new annotations are introduced to improve and adapt the existing dataset, ensuring a higher level of precision and relevance over time.
Data Annotation in SRDF begins with an initial labeling process, where human annotators or automated systems assign labels to data points. This step can cover various types of data, including text, images, audio, and video. To optimize this phase, specialized annotation algorithms are employed, ensuring accuracy across multiple data types. These algorithms use advanced machine learning methods to automate the majority of the labeling process, helping to scale it effectively.
However, automation alone is not enough. SRDF systems incorporate Quality Control (QC) Mechanisms to ensure that the data remains consistent and of high quality throughout the process. A combination of peer reviews, consistency checks, and feedback loops is essential for identifying and rectifying discrepancies. This iterative approach helps refine the annotations progressively, ensuring that the dataset evolves to better serve AI model training.
An important aspect of maintaining high-quality datasets within SRDF is addressing bias and noise. Automated labeling systems can inadvertently introduce biased labels, particularly when diverse data or edge cases are underrepresented. By integrating techniques like active learning, the SRDF system ensures a balanced representation of all classes, promoting fairness. Additionally, to tackle noise (irrelevant or erroneous labels), advanced data cleaning algorithms are used, which help identify and correct these issues before they affect model performance.
Finally, SRDF implements automated feedback loops, where model performance on labeled data is constantly evaluated. If a model performs poorly on certain data segments, those segments are flagged for re-annotation, ensuring a continual feedback-driven improvement. This mechanism not only increases the accuracy of the labeled data but also adapts to new patterns or shifts in data distribution.
In essence, SRDF combines state-of-the-art automated data labeling with meticulous quality control and feedback mechanisms, creating a self-improving system that enhances dataset quality over time, boosting the efficiency and performance of AI models.
To integrate feedback from model failures or inaccuracies, a Self-Refining Data Flywheel (SRDF) continuously improves both the dataset and model. This dynamic loop ensures that model performance evolves by learning from mistakes or mispredictions.
The first step in the SRDF involves collecting data from model outputs, especially from instances where the model fails or underperforms. By monitoring these failures, the system identifies specific weaknesses or patterns where the model struggles. Once the failures are identified, human annotators or automated systems label the problematic instances, providing corrections or additional context that the model initially missed.
After this, the feedback data is integrated back into the training dataset. This updated dataset is more representative of edge cases or areas that previously lacked sufficient training examples, allowing the model to understand complex or less frequent scenarios. By incorporating these refined data points, the SRDF system facilitates a model retraining cycle that enhances performance over time.
Moreover, this feedback loop works efficiently through active learning techniques, where the model selectively requests data points that are ambiguous or uncertain, which in turn enhances model precision by focusing on the most informative data. The cycle continues to grow, with each iteration improving model accuracy, especially on previously underrepresented examples.
In practice, these processes also involve automated tools that track model outputs and highlight discrepancies. As these systems identify failures, they initiate the process of correcting errors by re-training the model with new, refined data, which helps achieve a continually improving machine learning system.
By leveraging such a flywheel approach, datasets and models alike become more adaptive, continually refining each other to optimize the system's performance. This closed-loop mechanism ensures that the model learns not only from its successes but also from its failures, fostering sustainable improvement.
Advantages of SRDF for Vision-and-Language Navigation Datasets
SRDF, or Self-Refining Data Flywheel, plays a crucial role in addressing the common dataset limitations like data shift, annotation errors, and label inaccuracies that often hinder the quality of machine learning models, especially in vision-and-language navigation tasks. Let’s break down how SRDF helps tackle these challenges.
1. Mitigating Data Shift
Data shift refers to the changes in the distribution of data over time, which can negatively affect model accuracy if not properly handled. SRDF combats this issue by enabling continuous feedback loops within the dataset generation process. This means that as models are deployed and exposed to real-world data, SRDF dynamically adjusts the dataset to reflect emerging patterns and shifts in the data environment. As a result, the model is continuously updated with fresh and relevant data, minimizing the risks associated with data drift. For example, if a navigation model starts to underperform in certain environmental conditions, SRDF can automatically incorporate new data from similar conditions into the training set, ensuring that the model remains accurate even as the real world changes.
2. Addressing Annotation Errors
Annotation errors are a frequent challenge, especially when dealing with large datasets that require manual labeling. SRDF improves the annotation process by integrating AI-assisted labeling tools that help streamline the identification of errors during the annotation phase. These tools leverage machine learning models to detect discrepancies and inconsistencies in labels, such as misclassifications or missing data, which can then be flagged for human review. This not only reduces the burden on human annotators but also ensures that errors are minimized, leading to higher-quality datasets. Moreover, SRDF’s iterative approach means that it can gradually correct errors in a dataset by learning from past mistakes, leading to continual improvement in annotation accuracy.
3. Tackling Label Inaccuracies
Label inaccuracies can arise from several sources, including inconsistent labeling practices or incorrect data annotations. SRDF addresses this by using complex ontological structures that define clear relationships between data points, thus reducing the chances of mislabeled data. Additionally, SRDF employs a combination of active learning and expert review workflows. These methods ensure that labels are continually checked and refined, with mislabeling errors identified early in the process and corrected in real-time. The integration of AI-assisted labeling and feedback mechanisms allows for precise adjustments to the dataset, ensuring that the models receive high-quality labeled data, which is essential for training robust AI systems.
In conclusion, SRDF’s self-refining mechanisms provide a robust solution for overcoming common dataset issues like data shift, annotation errors, and label inaccuracies. By incorporating AI tools, continuous data feedback, and expert oversight, SRDF ensures that the datasets used for training vision-and-language models remain high-quality, relevant, and accurate over time. This is essential for advancing the field of AI research, where dataset quality directly impacts the success of machine learning models.
SRDF's role in improving model accuracy, particularly in complex environments where vision and language interact, is rooted in its ability to generate high-quality datasets that more effectively train vision-language models. Complex datasets, when structured appropriately, provide models with rich, diverse, and contextually accurate information, leading to better performance in interpreting nuanced visual and textual interactions. When training models for tasks that involve both visual data (like images or videos) and linguistic elements (such as descriptions or instructions), the accuracy of these models greatly depends on the quality of the training data.
High-quality datasets reduce noise and inaccuracies in both visual and textual data, ensuring that models learn correct associations between language and vision. This is particularly vital for tasks that require detailed understanding, such as navigation through environments based on both visual inputs (e.g., images) and textual commands. Poor-quality or inconsistent training data can introduce biases or distortions, which often results in lower model accuracy, especially in environments with dynamic or complex visual features. As a result, ensuring that datasets are well-labeled and representative of real-world scenarios is crucial for achieving high accuracy.
Technologies like Dynamic High Resolution, which ensures that high-resolution images are effectively processed, and CapFusion, which improves the syntactic and semantic structure of training captions, have shown that specific strategies in curating datasets can significantly improve model performance. Moreover, high-quality, carefully annotated data helps avoid common pitfalls like data bias, which can distort model predictions. By employing expert annotators and tools to maintain consistency and accuracy in dataset labeling, SRDF ensures that models trained on its datasets can interpret vision-and-language tasks with higher precision and lower error rates.
Real-World Examples
The use of SRDF (Structured Retrieval-augmented Deep Fusion) has demonstrated significant improvements in AI research, particularly in vision-and-language tasks. One notable implementation is in models like RAVEN (Retrieval-Augmented Vision-language Enhanced), which integrates retrieval techniques to enhance the capabilities of vision-language models. RAVEN improves tasks like image captioning and visual question answering (VQA) by retrieving relevant image-text pairs from external sources, such as large datasets like LAION-5B, and incorporating them into the model's responses. This leads to better task performance without requiring an expansion in the model's parameters, making it both efficient and scalable.
For example, in image captioning, RAVEN showed improvements on the MSCOCO dataset with a +1 CIDEr score increase, a significant gain given the complexity of the dataset's evaluation criteria. In VQA, it exhibited an accuracy increase of nearly 3% on specific question types, highlighting its capacity to handle complex visual queries more effectively. These case studies showcase how SRDF can be utilized to integrate external knowledge seamlessly, making models more adaptable and efficient, without the computational burden typically associated with increasing the model's size.
Furthermore, the RAVEN framework offers a multi-task learning approach that enhances performance across a variety of vision-language tasks, from captioning to more complex interactive visual question answering scenarios. By leveraging the retrieval of external context during processing, the model is able to make more accurate predictions based on a richer set of data. This method holds promise for scalable and sustainable AI development, addressing both the computational intensity and flexibility challenges often faced by traditional vision-language models.
These examples demonstrate that SRDF-based techniques like RAVEN are playing a crucial role in enhancing vision-language models by integrating external knowledge efficiently, contributing to advancements in AI research that require multitasking across vision, language, and retrieval domains.
Hasty, a leading platform in automated image annotation, offers robust tools for efficient labeling, ensuring high-quality datasets for AI model training. It provides automated labeling features where machine learning models can annotate images or datasets automatically. The system includes customizable parameters, such as confidence thresholds, to fine-tune the level of annotation certainty. These automated labels can be applied across an entire project or to specific parts of it, reducing the manual effort typically required in large-scale datasets.
Additionally, platforms like Hasty support AI-powered quality control and consistency features. For example, Hasty offers "AI Consensus Scoring," which evaluates the agreement between multiple AI-generated labels to ensure annotation quality. This feature helps to avoid errors, especially when dealing with complex datasets. Such quality control tools are integral to maintaining the precision of labeled data, which is essential for high-performing AI models in vision-and-language tasks.
These automated systems save researchers valuable time by allowing them to focus on higher-level tasks while the system handles repetitive annotation work. As AI datasets continue to grow, these tools help ensure that data labeling remains both scalable and accurate. Moreover, such platforms facilitate collaboration by enabling users to review and adjust annotations, ensuring a hybrid approach where AI assistance works in tandem with human oversight for the best results.
This combination of automation and quality control makes platforms like Hasty highly effective for creating the high-quality, large-scale datasets required in cutting-edge AI research, especially in vision-and-language navigation.
Challenges and Future Directions
Implementing the Semantic Research Data Framework (SRDF) in research environments presents a set of challenges, particularly related to data privacy and integration with existing research tools. Let's explore some of the critical concerns:
Data Privacy and Security: One of the primary obstacles is ensuring that sensitive data is adequately protected when implementing SRDF. AI-driven technologies, including SRDF, often require vast amounts of data to function efficiently. This creates privacy risks, as personal data or identifiable information may be inadvertently exposed during data analysis processes. The global landscape of privacy regulations, such as the GDPR in Europe and CCPA in the U.S., introduces compliance complexity, particularly when it comes to ensuring that collected data is anonymized or handled with explicit consent.
To mitigate these risks, SRDF implementations must adopt strict data governance and security measures. Data encryption, secure access controls, and continuous monitoring for privacy violations are essential. Moreover, ensuring that only necessary data is collected (data minimization) and employing AI-driven solutions for identifying sensitive information within datasets are best practices.
Integration with Existing Tools: Research environments often rely on a range of tools and databases that may not be fully compatible with SRDF. Integration issues can arise due to differing data structures, formats, or proprietary protocols used by existing research systems. Additionally, if SRDF requires the overhaul of existing data management practices, it can lead to resistance from researchers who are accustomed to their current workflows.
For example, a major hurdle in adopting SRDF could involve overcoming siloed data storage systems or mismatches in how various tools interpret and use semantic metadata. Successful integration would likely require significant collaboration between developers of SRDF and the developers of existing research tools to establish standardized interfaces and protocols.
Scalability and Performance: As SRDF can handle vast datasets, scaling it to meet the needs of large-scale research projects is another challenge. The volume of data used in academic research continues to grow exponentially, and managing this data efficiently while maintaining performance can be difficult. Optimizing SRDF for performance without compromising data integrity or privacy becomes a balancing act. Additionally, the AI algorithms used to analyze this data must be fine-tuned to minimize resource consumption, as the tools often require significant computational power.
Adapting to Evolving Legal and Ethical Standards: As AI technologies evolve rapidly, so too do the legal and ethical standards surrounding data privacy. SRDF implementations must be flexible enough to adapt to these evolving regulations. For instance, new policies might require more granular user consent processes or adjustments in how data is shared and accessed, and SRDF frameworks need to incorporate these requirements proactively.
To address these challenges effectively, it is crucial to establish dedicated teams that can focus on navigating both technical and regulatory complexities. By ensuring that SRDF aligns with both privacy laws and the needs of existing tools, its adoption can be more seamless and beneficial to researchers.
As SRDF (Semantic Representation Data Format) continues to evolve, its applications are likely to extend to more complex datasets and AI models, thanks to several advancements in technology. The evolution of SRDF in AI will be shaped by its ability to manage and utilize larger datasets, which is essential as AI models become more sophisticated. One major aspect of this progression will involve handling the increasingly complex nature of datasets, such as those generated in fields like genomics, autonomous vehicles, and personalized healthcare. These datasets often contain diverse, high-dimensional data that SRDF could optimize for both storage and processing efficiency.
One key trend in AI is the growing need for memory and storage solutions that can support large-scale, real-time processing at the edge. This includes advancements in embedded systems that allow AI models to perform computations directly on local devices rather than relying solely on cloud infrastructure. These systems need faster and more efficient memory solutions, like LPDDR5 and NVMe SSDs, which help meet the high demands of complex AI models. With such technologies, SRDF could evolve to manage datasets in ways that balance the requirements for speed, storage, and real-time computation, improving both the efficiency and accuracy of AI-driven tasks.
The future of SRDF could also see the adoption of distributed architectures, such as those found in edge computing systems. By processing data closer to where it’s generated, SRDF could help reduce latency and increase the responsiveness of AI models, a significant advantage in fields like autonomous driving, where split-second decisions are required. This also ties into the potential for SRDF to support AI models that require continuous learning or adaptation, which are becoming more common in dynamic environments like financial trading or healthcare diagnostics.
Looking ahead, SRDF's integration into the broader ecosystem of machine learning will also be crucial. As machine learning models expand and incorporate more complex algorithms with a growing number of parameters, SRDF will need to scale to accommodate these changes. Advances in data architecture and AI chipsets—like AI processors optimized for edge computing—are pushing SRDF’s potential to manage diverse, high-volume data more efficiently. These advancements will be key to unlocking more powerful and scalable AI applications.
Conclusion
The value of Structured Recursive Data Framework (SRDF) in advancing AI research lies primarily in its capacity to refine and optimize datasets for more efficient model training. SRDF facilitates the processing and organization of vast datasets, which is crucial for developing AI models that can perform with high accuracy and reliability. The core advantage of SRDF is its ability to break down complex data into structured, recursive components, making it easier for machine learning algorithms to identify patterns and relationships within the data. This process is pivotal for improving AI decision-making, adaptability, and the generalization capabilities of models.
By refining datasets in this way, SRDF directly contributes to increasing the performance of AI models, ensuring they are better equipped to handle diverse, real-world scenarios. For instance, in fields like healthcare, finance, or cybersecurity, where vast and varied datasets are involved, SRDF can help build models that are more robust and capable of making informed decisions under uncertainty. Furthermore, SRDF enhances the quality of AI predictions by reducing noise and irrelevant data, which is essential for tasks such as predictive maintenance, fraud detection, and customer personalization.
In the broader context, SRDF's role is vital for ensuring that AI models are not only accurate but also scalable and adaptable to new data inputs. This makes it a powerful tool for businesses and researchers looking to harness the full potential of AI. Moreover, the integration of SRDF into the data pipeline supports continuous learning, allowing AI systems to evolve and improve over time as new data becomes available. This creates a feedback loop that helps maintain the relevance and precision of AI models.
In summary, SRDF serves as a foundational technology that enables better dataset management, which is essential for training more accurate, adaptable, and efficient AI models. As industries continue to rely on AI for advanced decision-making and automation, SRDF's role in refining datasets will only grow, leading to more powerful AI systems that can drive innovation across multiple sectors.
To integrate SRDF (Self-Refining Data Flywheel) into your own research workflows, it's essential to understand its core functionality and how it can streamline data handling in AI projects, particularly in vision-and-language navigation tasks. SRDF helps improve dataset quality over time by leveraging a cycle of continuous feedback and data refinement. Researchers can employ SRDF to address common challenges in dataset construction and maintenance, especially in tasks that require high accuracy and domain specificity.
The self-refining nature of SRDF means that it not only provides high-quality data initially but also enhances its own quality as more data is processed, reducing the need for manual interventions and improving the reliability of machine learning models over time. This is particularly valuable in areas where annotated datasets are scarce or difficult to obtain.
To incorporate SRDF into your workflow, start by ensuring that your existing datasets can be easily fed into SRDF's automated data improvement system. This integration involves using APIs or system interfaces to allow for seamless communication between your research tools and SRDF. Once set up, SRDF will automatically refine the data, providing researchers with continually improving datasets that are aligned with their specific needs. This will free up time and resources, enabling researchers to focus more on model development and less on the tedious task of data collection and curation.
Additionally, SRDF's ability to dynamically adapt to new data and feedback can foster collaboration across different research teams and projects, allowing them to build on each other's datasets and improving the generalizability of AI models. By integrating SRDF, you can reduce the time it takes to develop high-quality AI systems, while also contributing to a more sustainable and efficient research ecosystem.
Researchers in fields such as computer vision, natural language processing, and robotics can particularly benefit from SRDF’s capabilities. Given the challenges of acquiring high-quality, annotated datasets, SRDF offers a robust solution that can help to optimize the process, making it more cost-effective and less time-consuming.
For further exploration, consider looking into how SRDF fits within the broader landscape of automated data processing and AI dataset strategies. Engaging with the growing body of research on SRDF can provide valuable insights into its full potential and how it can be adapted to various research environments.
Press contact
Timon Harz
oneboardhq@outlook.com
Other posts
Company
About
Blog
Careers
Press
Legal
Privacy
Terms
Security