Timon Harz
December 18, 2024
Infinigence AI Launches Megrez-3B-Omni: Open-Source 3B Multimodal LLM for On-Device Use
Megrez-3B-Omni combines cutting-edge AI technologies to enhance on-device processing, offering faster, more private solutions. Its versatility across text, speech, and image understanding makes it a game-changer for various applications.
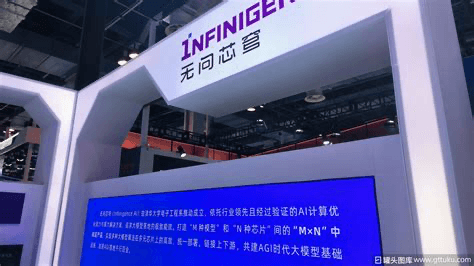
Integrating artificial intelligence into everyday life comes with significant challenges, especially in multimodal understanding—the ability to process and analyze text, audio, and visual inputs. Many AI models depend on powerful cloud-based infrastructure, requiring substantial computational resources. This reliance introduces issues like latency, energy inefficiency, and data privacy concerns, which hinder deployment on devices such as smartphones or IoT systems. Moreover, balancing performance across multiple modalities often requires trade-offs in accuracy or efficiency. These obstacles have driven the development of solutions that are both lightweight and highly effective.
Megrez-3B-Omni: A 3B On-Device Multimodal LLM
Infinigence AI presents Megrez-3B-Omni, a 3-billion-parameter on-device multimodal large language model (LLM). Building on their Megrez-3B-Instruct framework, this model processes text, audio, and image inputs simultaneously. Unlike traditional cloud-based models, Megrez-3B-Omni focuses on on-device operation, offering low latency, strong privacy, and efficient resource usage. Designed for resource-constrained devices, it aims to make advanced AI technology more accessible, practical, and user-friendly.
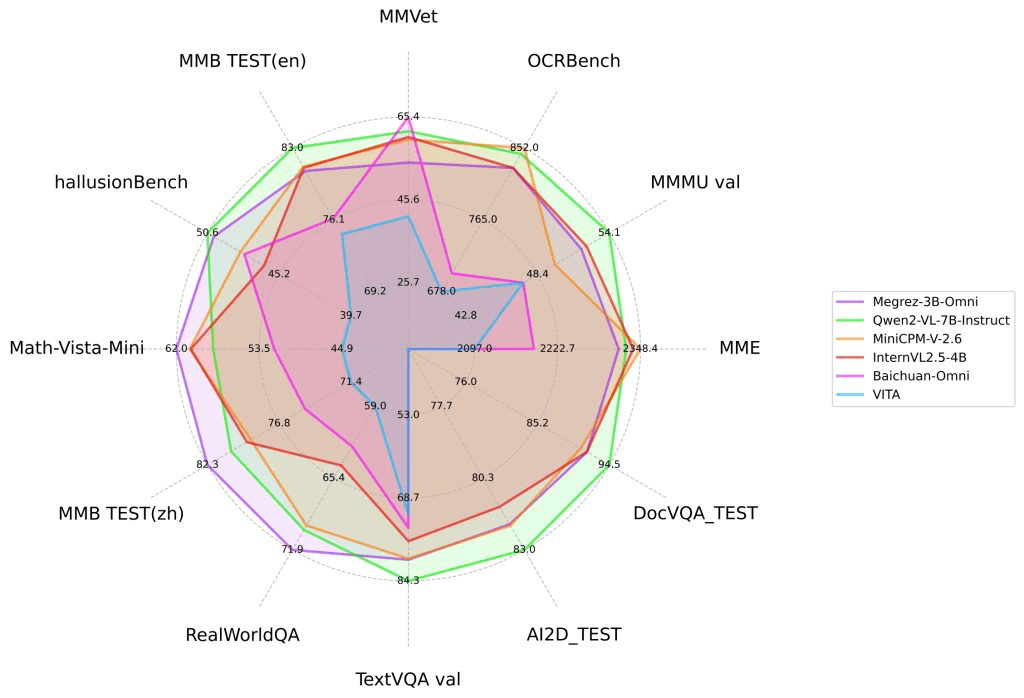
Technical Details
Megrez-3B-Omni includes several advanced technical features that improve its performance across multiple modalities. Central to its design is the use of SigLip-400M for constructing image tokens, which enhances its ability to understand images. This allows the model to excel in tasks like scene interpretation and optical character recognition (OCR), outperforming larger models, such as LLaVA-NeXT-Yi-34B, on benchmarks like MME, MMMU, and OCRBench.
For language processing, Megrez-3B-Omni delivers high accuracy with minimal trade-offs when compared to its unimodal predecessor, Megrez-3B-Instruct. Benchmark tests, including C-EVAL, MMLU/MMLU Pro, and AlignBench, validate its strong language performance.
For speech processing, the model integrates the encoder head from Qwen2-Audio/whisper-large-v3, enabling it to process both Chinese and English speech. It supports multi-turn conversations and voice-based queries, unlocking new possibilities for interactive applications, such as voice-activated visual searches and real-time transcription. This seamless integration of text, speech, and image modalities enhances its practical value in scenarios where multiple inputs converge.
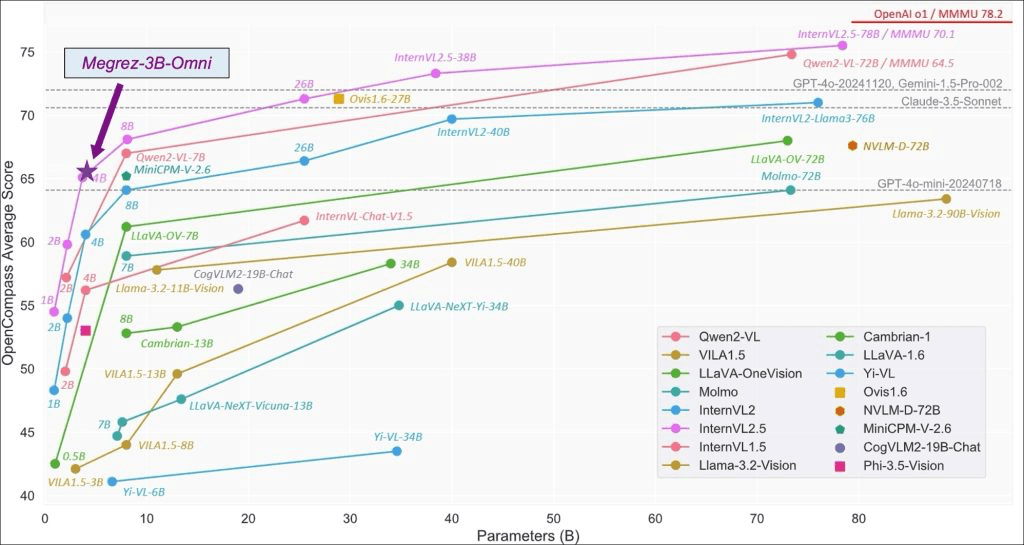
Results and Performance Insights
Megrez-3B-Omni delivers impressive results across key benchmarks, showcasing its strengths in multimodal tasks. In image understanding, it consistently outperforms larger models in areas like scene recognition and OCR. For text analysis, it maintains high accuracy across both English and Chinese benchmarks, matching the performance of its unimodal predecessor.
In speech processing, Megrez-3B-Omni excels in bilingual contexts, effectively handling tasks involving voice input and text responses. Its ability to manage natural, multi-turn dialogues makes it an attractive option for conversational AI applications. Comparisons with older, larger models highlight its superior efficiency and effectiveness.
The model's on-device operation further sets it apart, removing the need for cloud-based processing. This reduces latency, enhances privacy, and lowers operational costs. These features make Megrez-3B-Omni particularly valuable in fields such as healthcare and education, where secure and efficient multimodal analysis is essential.
Megrez-3B-Omni marks a significant step forward in multimodal AI. By integrating strong performance across text, audio, and image modalities with an efficient on-device architecture, the model addresses critical challenges related to scalability, privacy, and accessibility. Its results across various benchmarks prove that high performance doesn't have to compromise efficiency or usability. As multimodal AI continues to evolve, Megrez-3B-Omni sets a practical example of how advanced AI can be seamlessly integrated into everyday devices, driving broader adoption of AI technologies.
Infinigence AI has introduced Megrez-3B-Omni, an open-source, on-device multimodal large language model (LLM) designed to process text, audio, and images. This 3-billion-parameter model builds upon the Megrez-3B-Instruct framework, enhancing its capabilities to handle diverse data types directly on user devices.
Unlike traditional cloud-dependent models, Megrez-3B-Omni operates entirely on-device, offering significant advantages in latency reduction, privacy enhancement, and resource efficiency. By processing data locally, it minimizes the need for cloud-based computations, thereby improving response times and safeguarding user data.
The model's multimodal proficiency is achieved through the integration of SigLip-400M for image token construction, enabling advanced image understanding capabilities. This allows Megrez-3B-Omni to excel in tasks such as scene comprehension and optical character recognition (OCR), outperforming models with larger parameter counts on various benchmarks.
In the realm of language processing, Megrez-3B-Omni maintains high accuracy with minimal trade-offs compared to its unimodal predecessor. It demonstrates strong performance on benchmarks like C-EVAL, MMLU/MMLU Pro, and AlignBench, showcasing its robust text understanding capabilities.
For speech understanding, the model incorporates the encoder head of Qwen2-Audio/whisper-large-v3, enabling it to process both Chinese and English speech inputs. This integration supports multi-turn conversations and voice-based queries, broadening its applicability in interactive applications such as voice-activated visual searches and real-time transcription.
By combining these advanced features, Megrez-3B-Omni sets a new standard for on-device multimodal AI models, offering a versatile and efficient solution for a wide range of applications.
On-device AI offers several significant advantages that enhance user experience and operational efficiency. By processing data directly on the device, it ensures improved privacy and data security, as sensitive information does not need to be transmitted over networks. This local processing also reduces latency, providing faster responses and more seamless interactions. Additionally, on-device AI decreases reliance on cloud services, leading to cost savings and reduced network congestion. These benefits make on-device AI particularly valuable in applications where privacy, speed, and efficiency are paramount.
Technical Details
Megrez-3B-Omni is an on-device multimodal large language model (LLM) developed by Infinigence AI, featuring 3 billion parameters. This model is an extension of the Megrez-3B-Instruct framework, designed to process and understand text, audio, and image inputs simultaneously.
A key component of Megrez-3B-Omni's architecture is the integration of SigLip-400M, a vision encoder that constructs image tokens. This integration enables the model to perform advanced image understanding tasks, such as scene comprehension and optical character recognition (OCR), with remarkable efficiency. Notably, Megrez-3B-Omni outperforms models with significantly larger parameter counts, including LLaVA-NeXT-Yi-34B, on benchmarks like MME, MMMU, and OCRBench.
In the realm of language processing, Megrez-3B-Omni maintains high accuracy with minimal trade-offs compared to its unimodal predecessor. It demonstrates strong performance on benchmarks such as C-EVAL, MMLU/MMLU Pro, and AlignBench, showcasing its robust text understanding capabilities.
For speech understanding, the model incorporates the encoder head of Qwen2-Audio/whisper-large-v3, enabling it to process both Chinese and English speech inputs. This integration supports multi-turn conversations and voice-based queries, broadening its applicability in interactive applications such as voice-activated visual searches and real-time transcription.
By combining these advanced features, Megrez-3B-Omni sets a new standard for on-device multimodal AI models, offering a versatile and efficient solution for a wide range of applications.
Megrez-3B-Omni is an on-device multimodal large language model (LLM) developed by Infinigence AI, designed to process and understand text, audio, and image inputs. This model extends the Megrez-3B-Instruct framework, integrating advanced components to handle diverse data types effectively.
For image understanding, Megrez-3B-Omni utilizes SigLip-400M to construct image tokens, enabling the model to perform tasks such as scene comprehension and optical character recognition (OCR). This integration allows the model to achieve state-of-the-art performance on benchmarks like MME, MMMU, and OCRBench, surpassing models with larger parameter counts, including LLaVA-NeXT-Yi-34B.
In language processing, Megrez-3B-Omni maintains high accuracy with minimal trade-offs compared to its unimodal predecessor. It demonstrates strong performance on benchmarks such as C-EVAL, MMLU/MMLU Pro, and AlignBench, showcasing its robust text understanding capabilities.
For speech understanding, the model incorporates the encoder head of Qwen2-Audio/whisper-large-v3, enabling it to process both Chinese and English speech inputs. This integration supports multi-turn conversations and voice-based queries, broadening its applicability in interactive applications such as voice-activated visual searches and real-time transcription.
By combining these advanced features, Megrez-3B-Omni sets a new standard for on-device multimodal AI models, offering a versatile and efficient solution for a wide range of applications.
Performance and Benchmarks
Megrez-3B-Omni, developed by Infinigence AI, demonstrates exceptional performance in image-related tasks, including scene understanding and optical character recognition (OCR). By utilizing SigLip-400M for constructing image tokens, the model outperforms larger models such as LLaVA-NeXT-Yi-34B across multiple mainstream benchmarks, including MME, MMMU, and OCRBench.
In contrast, LLaVA-NeXT-Yi-34B, while a significant advancement in multimodal AI, has not been reported to surpass Megrez-3B-Omni in these specific image understanding benchmarks. LLaVA-NeXT-Yi-34B has demonstrated strong performance in various multimodal understanding tasks, including OCR, but Megrez-3B-Omni's integration of SigLip-400M for image token construction provides it with a distinct advantage in image-related tasks.
This superior performance underscores Megrez-3B-Omni's capability to effectively process and understand visual information, making it a valuable tool for applications requiring advanced image comprehension.
Megrez-3B-Omni, developed by Infinigence AI, demonstrates exceptional performance in text understanding, maintaining high accuracy across various benchmarks. When evaluated on C-EVAL, MMLU/MMLU Pro, and AlignBench, the model exhibits minimal accuracy variation—less than 2%—compared to its unimodal predecessor, Megrez-3B-Instruct. This consistency underscores its robust language processing capabilities.
Notably, Megrez-3B-Omni outperforms previous-generation models with 14 billion parameters, highlighting its efficiency and effectiveness in language understanding tasks.
These results position Megrez-3B-Omni as a leading model in language processing, offering reliable and accurate text understanding across multiple benchmarks.
Megrez-3B-Omni, developed by Infinigence AI, excels in processing speech inputs, supporting both Chinese and English languages. Equipped with the encoder head of Qwen2-Audio/whisper-large-v3, the model effectively handles multi-turn conversations and voice-based queries, enabling interactive applications such as voice-activated visual searches and real-time transcription.
This integration allows Megrez-3B-Omni to directly respond to voice commands with text, achieving leading results across multiple benchmarks.
By combining these advanced features, Megrez-3B-Omni sets a new standard for on-device multimodal AI models, offering a versatile and efficient solution for a wide range of applications.
Applications and Use Cases
Megrez-3B-Omni's on-device processing capabilities offer significant advantages across various sectors, including healthcare, education, and personal assistance.
In healthcare, AI-powered virtual assistants can collect basic patient information through voice or text interactions, reducing the need for manual data entry and minimizing errors. This streamlines the intake process and allows medical staff to focus on patient interaction and building rapport.
In education, cognitive computing can be applied by having an assistant personalized for each individual student. This cognitive assistant can relieve the stress that teachers face while teaching students, while also enhancing the student's learning experience overall.
For personal assistants, AI-powered virtual assistants are virtual software agents designed to assist users with various tasks and provide information using artificial intelligence.
By leveraging on-device processing, these applications can operate efficiently without relying on cloud services, ensuring faster response times and enhanced privacy for users.
On-device AI significantly enhances data privacy and security by processing information directly on user devices, thereby minimizing the need for cloud-based data handling. This approach ensures that sensitive data, such as personal communications and health records, remain on the device, reducing the risk of unauthorized access during transmission. By keeping data on the device, users can enjoy AI-driven features without exposing their information to external servers.
Additionally, on-device AI reduces latency, providing instant results and eliminating the delays associated with cloud-based computations.
Furthermore, on-device AI can enable private and secure travel guidance from your digital assistant that is personalized based on contextual information.
By processing data locally, on-device AI offers a more secure and efficient alternative to traditional cloud-based AI solutions.
Conclusion
Megrez-3B-Omni, developed by Infinigence AI, significantly enhances the accessibility and practicality of advanced AI capabilities for everyday use. By integrating multimodal understanding—processing text, audio, and images—into a single on-device model, it enables a wide range of applications without the need for cloud-based processing. This approach reduces latency, enhances privacy, and minimizes reliance on external servers, making sophisticated AI functionalities more accessible to users across various devices.
The model's open-source nature further democratizes access to advanced AI technologies, allowing developers and researchers to customize and deploy it across diverse platforms. This flexibility fosters innovation and facilitates the integration of AI into various sectors, including healthcare, education, and personal assistance, thereby broadening the scope of AI applications in daily life.
The advent of on-device multimodal AI models, exemplified by Megrez-3B-Omni, signifies a transformative shift across various industries. By processing text, audio, and images directly on devices, these models enhance efficiency, reduce latency, and bolster data privacy.
In healthcare, on-device AI can streamline patient intake by collecting information through voice or text interactions, minimizing manual data entry and errors. This efficiency allows medical staff to focus more on patient care.
In education, AI-powered assistants can personalize learning experiences for students, alleviating teacher workload and enhancing the overall learning process.
For personal assistants, AI-powered virtual assistants are virtual software agents designed to assist users with various tasks and provide information using artificial intelligence.
The integration of on-device AI models like Megrez-3B-Omni is poised to revolutionize industries by enabling real-time, context-aware applications that enhance user experiences and operational efficiencies. As these technologies evolve, they will continue to drive innovation and transform various sectors.
Press contact
Timon Harz
oneboardhq@outlook.com
Other posts
Company
About
Blog
Careers
Press
Legal
Privacy
Terms
Security