Timon Harz
December 23, 2024
Hugging Face Launches FineMath: The Ultimate Open Math Pre-Training Dataset with 50B+ Tokens
FineMath by Hugging Face sets a new standard in open-source mathematical datasets, empowering learners and researchers with high-quality resources. Its focus on transparency and accessibility revolutionizes AI-driven education.
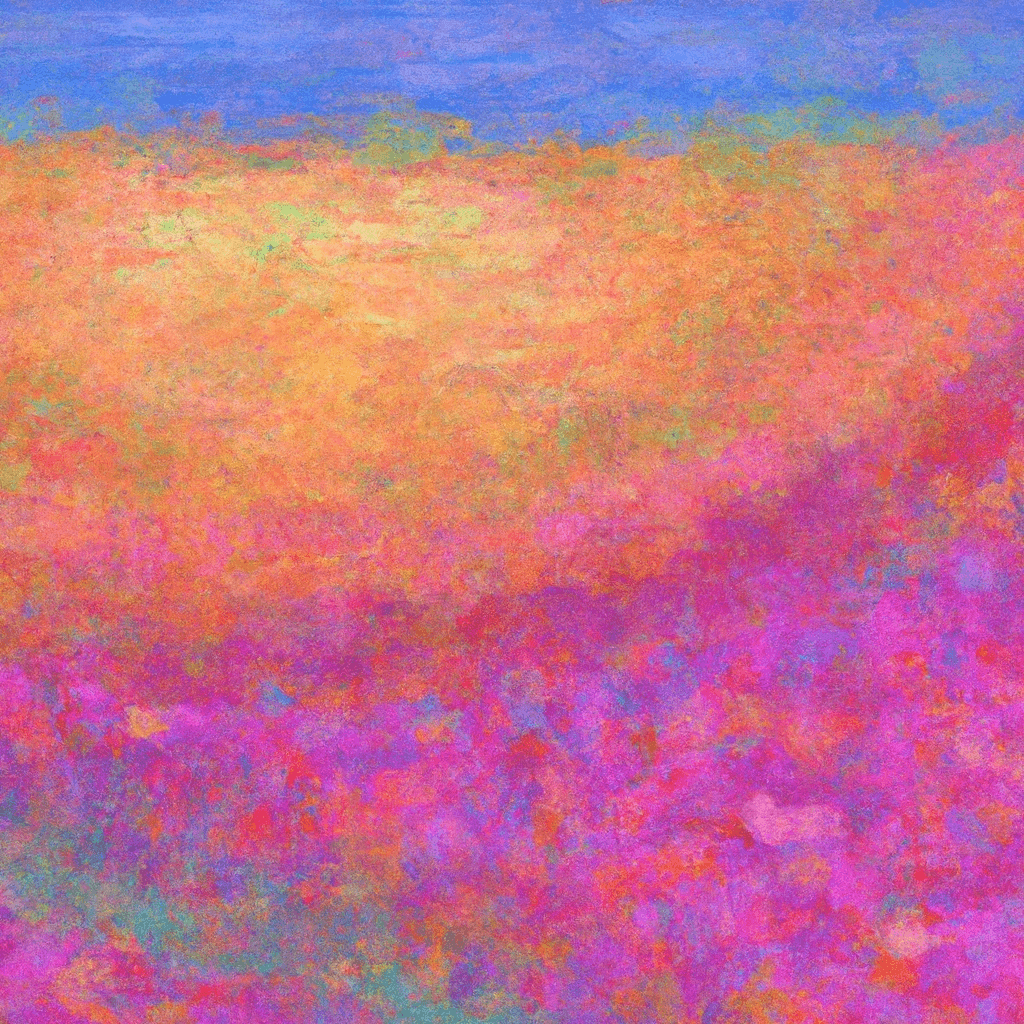
Access to high-quality educational resources is essential for both learners and educators, especially in fields like mathematics, which is often seen as one of the most challenging subjects. Clear explanations and well-structured resources are crucial for effective learning. However, creating and curating datasets specifically focused on mathematical education presents significant challenges. Many datasets used to train machine learning models are proprietary, limiting transparency in how educational content is selected, structured, and optimized. The lack of open-source datasets for mathematics hinders the development of AI-driven educational tools.
To address these challenges, Hugging Face has launched FineMath, a groundbreaking initiative aimed at providing open access to high-quality mathematical content for learners and researchers alike. FineMath is a comprehensive dataset designed specifically for mathematical education and reasoning. It tackles the difficulties of sourcing, curating, and refining mathematical content from diverse online sources. The dataset is carefully constructed to support machine learning models focused on mathematical problem-solving and reasoning tasks.
FineMath comes in two main versions:
FineMath-3+: Comprising 34 billion tokens from 21.4 million documents, formatted in Markdown and LaTeX to preserve mathematical integrity.
FineMath-4+: A subset of FineMath-3+, with 9.6 billion tokens across 6.7 million documents, focusing on higher-quality content with more detailed explanations.
These carefully curated subsets ensure that both general learners and advanced models can benefit from FineMath’s robust framework.
The creation of FineMath involved a multi-phase process to efficiently extract and refine the content. The process began with raw data extraction from CommonCrawl, using advanced tools like Resiliparse to capture text and formatting precisely. A custom classifier based on Llama-3.1-70B-Instruct was then used to evaluate the dataset, scoring pages based on logical reasoning and the clarity of step-by-step solutions. The dataset was further expanded while maintaining high quality, addressing challenges such as improper LaTeX notation handling, and ensuring better preservation of mathematical expressions. Deduplication and multilingual evaluation were also conducted to enhance the dataset’s relevance and usability.
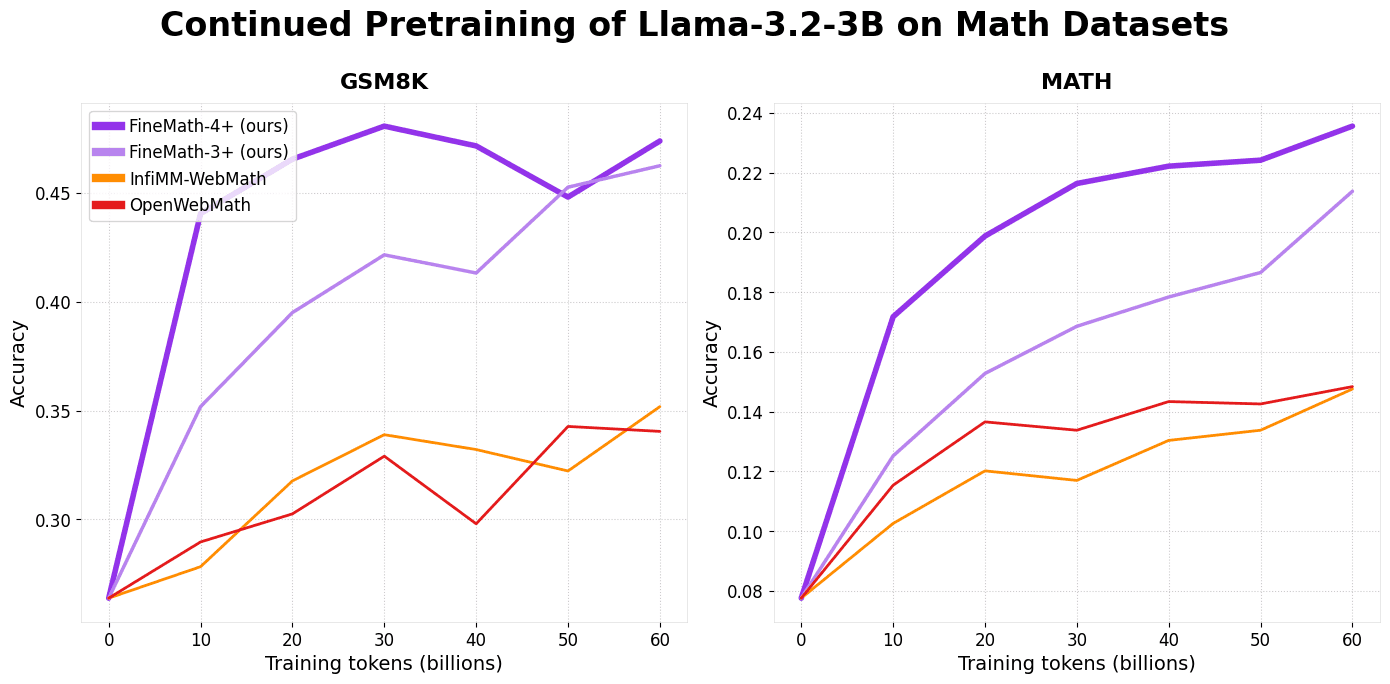
FineMath has shown exceptional performance on well-established benchmarks like GSM8k and MATH, with models trained on FineMath-3+ and FineMath-4+ demonstrating significant improvements in mathematical reasoning and accuracy. By combining FineMath with other datasets, such as InfiMM-WebMath, researchers can create a larger dataset of approximately 50 billion tokens, all while maintaining outstanding performance. FineMath’s structure is designed for seamless integration into machine learning pipelines. With Hugging Face’s comprehensive library support, developers can easily load subsets of the dataset, facilitating experimentation and deployment across a wide range of educational AI applications.
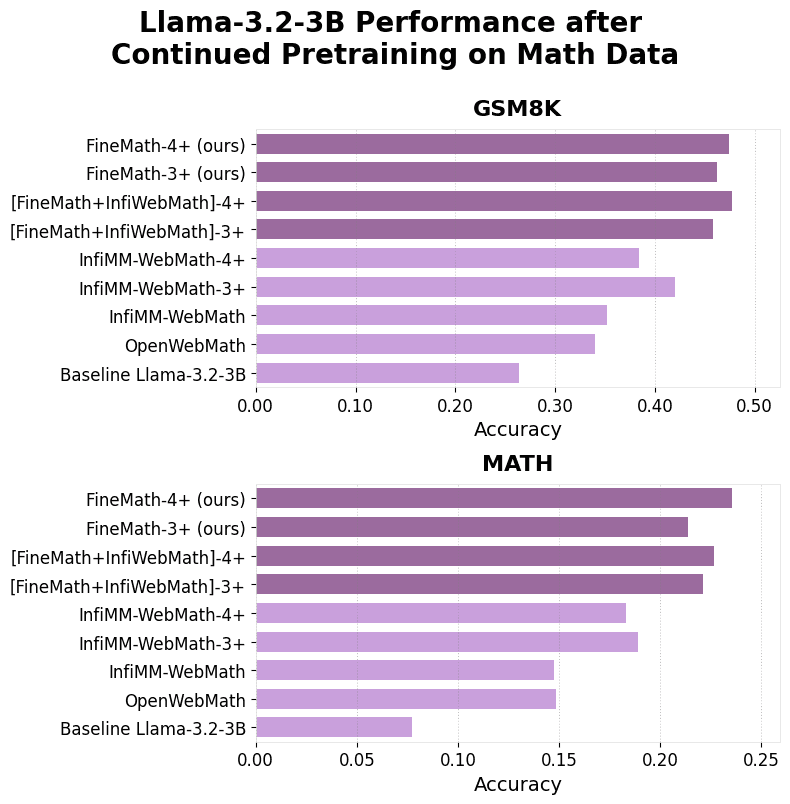
In conclusion, Hugging Face’s FineMath dataset is a groundbreaking contribution to both mathematical education and AI. By addressing gaps in accessibility, quality, and transparency, it sets a new standard for open educational resources. Future developments for FineMath include expanding language support beyond English, improving mathematical notation extraction and preservation, refining quality metrics, and creating specialized subsets for various educational levels.
Press contact
Timon Harz
oneboardhq@outlook.com
Other posts
Company
About
Blog
Careers
Press
Legal
Privacy
Terms
Security