Timon Harz
December 17, 2024
DeepSeek-AI Open Sources DeepSeek-VL2 Series: 3B, 16B, and 27B Parameter Models with Mixture-of-Experts (MoE) Architecture Transforming Vision-Language AI
The DeepSeek-VL2 series marks a new era in vision-language AI, enabling more accurate and efficient solutions across various sectors. Discover how these powerful models are transforming the way we interact with visual and textual data.
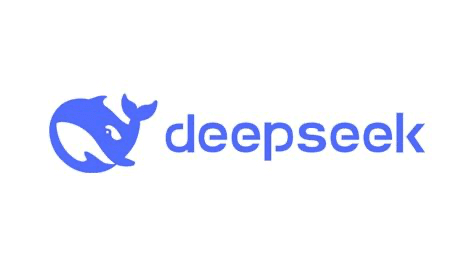
The integration of vision and language in AI has driven significant advances in Vision-Language Models (VLMs). These models are designed to process and interpret both visual and textual data simultaneously, enabling a wide range of applications such as image captioning, visual question answering, optical character recognition, and multimodal content analysis. VLMs are crucial for the development of autonomous systems, improving human-computer interactions, and creating more efficient document processing tools by bridging the gap between visual and textual data. However, handling high-resolution visual inputs alongside diverse textual data remains a significant challenge in this field.
Previous research has attempted to address these challenges with static vision encoders, but these models often lack the flexibility needed to handle varying input sizes and high-resolution data. Pretrained language models, when used with vision encoders, can introduce inefficiencies since they are typically not optimized for multimodal tasks. Some models have tried incorporating sparse computation techniques to manage complexity, but they still struggle to deliver consistent accuracy across different datasets. Additionally, the training datasets used in these models often lack sufficient diversity and task-specific granularity, limiting their performance. As a result, many models still underperform in specialized tasks, such as chart interpretation or dense document analysis, due to these limitations.
Researchers at DeepSeek-AI have unveiled the DeepSeek-VL2 series, a next-generation collection of open-source mixture-of-experts (MoE) vision-language models. These models incorporate advanced features such as dynamic tiling for vision encoding, a Multi-head Latent Attention mechanism for language tasks, and the DeepSeek-MoE framework. The DeepSeek-VL2 series offers three configurations, each with varying numbers of activated parameters (activated parameters refer to the subset of a model's parameters that are dynamically used during a specific task or computation):
DeepSeek-VL2-Tiny: 3.37 billion total parameters (1.0 billion activated parameters)
DeepSeek-VL2-Small: 16.1 billion total parameters (2.8 billion activated parameters)
DeepSeek-VL2: 27.5 billion total parameters (4.5 billion activated parameters)
This scalability provides flexibility, making the models suitable for a wide range of applications and computational resources.
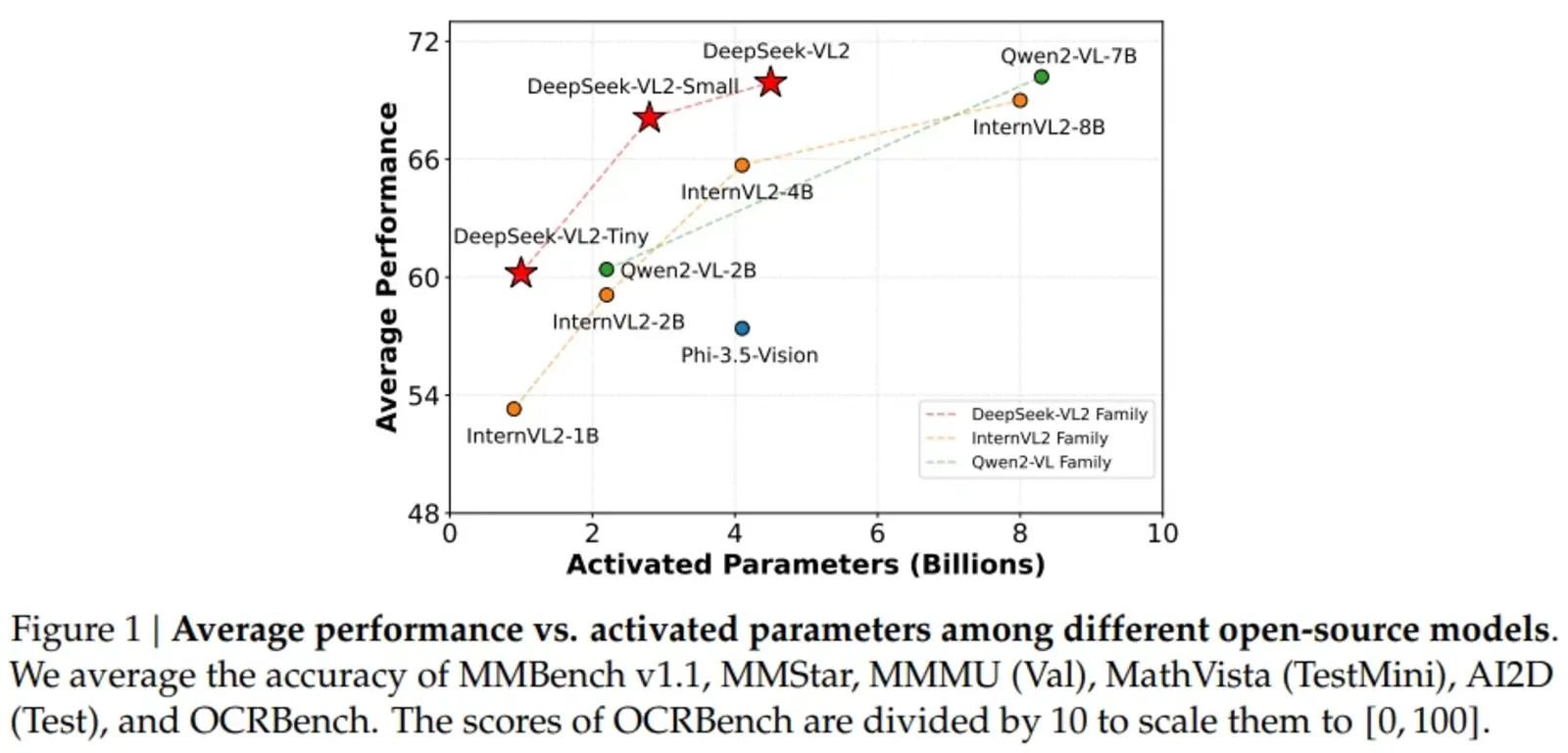
The DeepSeek-VL2 architecture is specifically designed to deliver high performance while minimizing computational requirements. The dynamic tiling technique ensures that high-resolution images are processed with full detail, making it particularly effective for tasks like document analysis and visual grounding. Additionally, the Multi-head Latent Attention mechanism enables the model to efficiently handle large amounts of textual data, reducing the computational burden typically associated with processing complex language inputs. The DeepSeek-MoE framework, which activates only a subset of parameters during task execution, further boosts scalability and efficiency. DeepSeek-VL2’s training uses a rich and diverse multimodal dataset, allowing the model to perform exceptionally well in a variety of tasks, including optical character recognition (OCR), visual question answering, and chart interpretation.
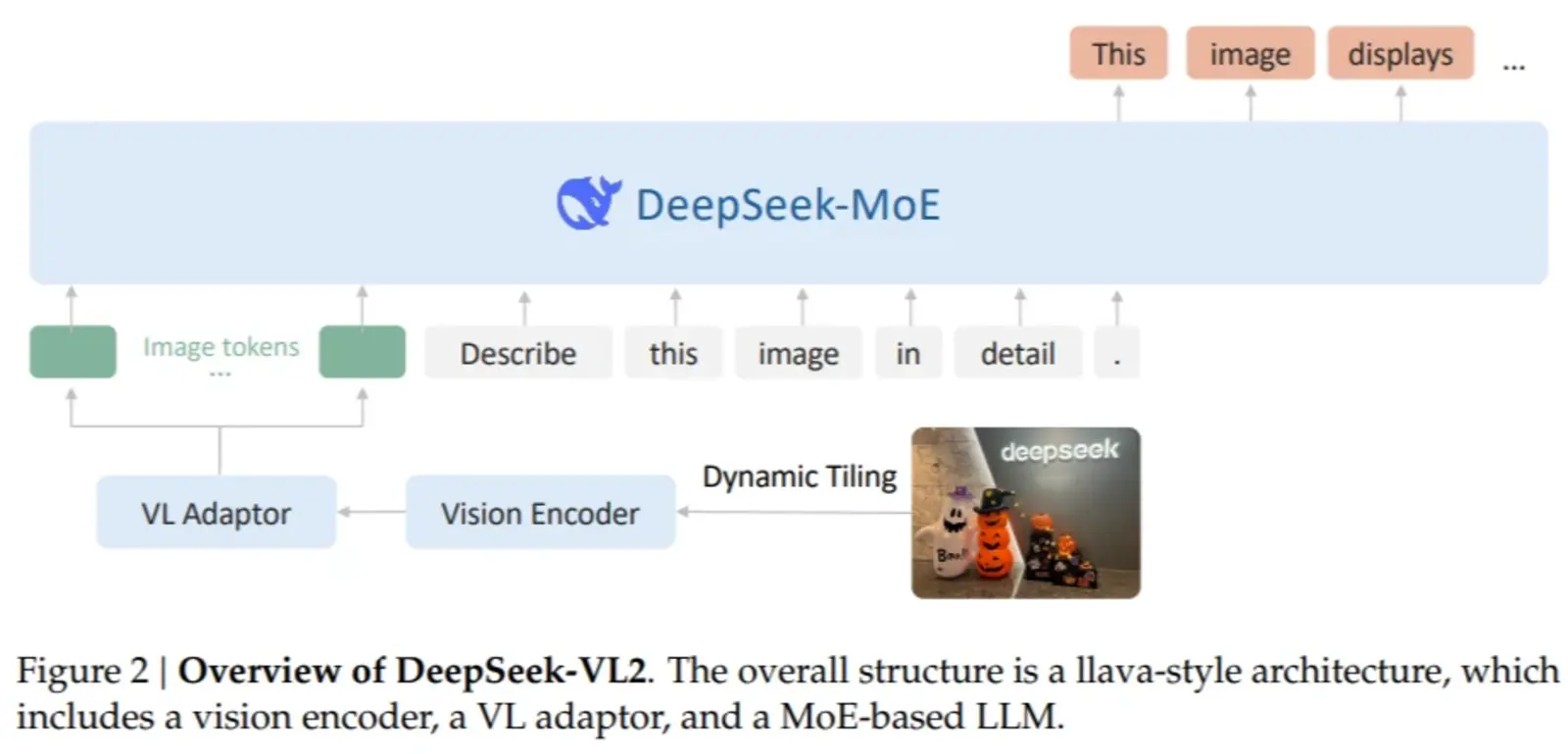
During performance testing, the DeepSeek-VL2 small configuration achieved an impressive 92.3% accuracy on OCR tasks, surpassing existing models by a significant margin. In visual grounding benchmarks, it demonstrated a 15% improvement in precision over previous models. Additionally, DeepSeek-VL2 showcased exceptional efficiency, requiring 30% less computational power than comparable models, all while maintaining state-of-the-art accuracy. The model's ability to generalize across tasks was also evident, with its Standard variant delivering top scores in multimodal reasoning benchmarks. These results highlight the model’s effectiveness in overcoming the challenges of high-resolution image and text processing.
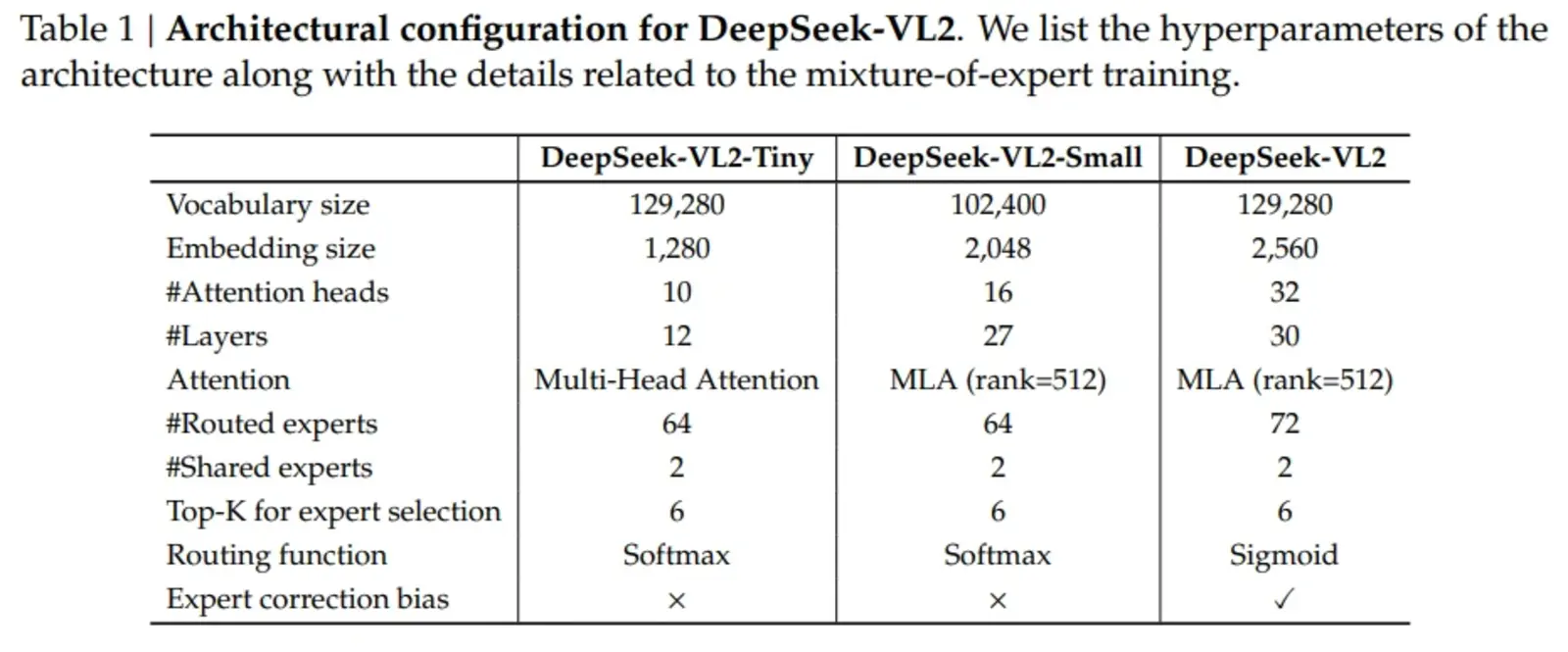
Key takeaways from the DeepSeek-VL2 model series include:
The use of dynamic tiling to divide high-resolution images into smaller segments enhances feature extraction and reduces computational overhead, making it ideal for dense document analysis and intricate visual layouts.
The availability of configurations with tiny (3B), small (16B), and standard (27B) parameters provides flexibility, supporting a wide range of applications from lightweight deployments to resource-intensive tasks.
The model’s comprehensive training dataset, which includes OCR and visual grounding tasks, boosts its generalizability and performance across various specialized tasks.
The sparse computation framework activates only the necessary parameters, reducing computational costs while maintaining high accuracy.
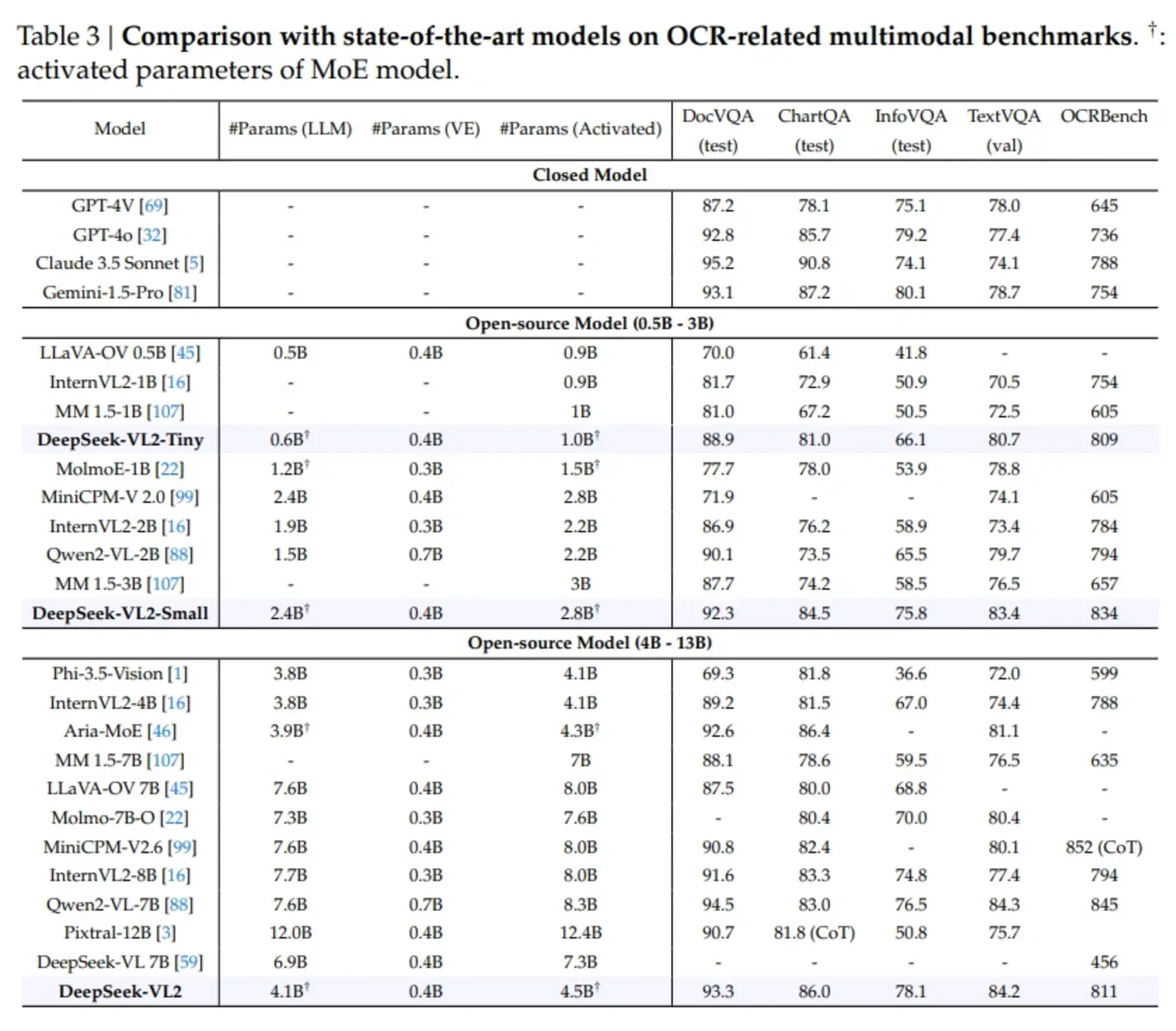
In conclusion, the DeepSeek-VL2 series is an open-source vision-language model with three variants, offering 1.8B, 2.8B, and 4.5B activated parameters. The research team has developed a model series that excels in real-world applications by overcoming key challenges in scalability, computational efficiency, and task adaptability. With its innovative dynamic tiling and Multi-head Latent Attention mechanisms, the models achieve precise image processing and efficient text handling, delivering state-of-the-art performance in tasks such as OCR and visual grounding. This series sets a new benchmark in AI, offering scalable configurations and leveraging a comprehensive multimodal dataset to push the boundaries of AI performance.
DeepSeek-AI, established in 2023, has rapidly emerged as a prominent force in the artificial intelligence landscape. Founded by Liang Wenfeng, a former executive at High-Flyer Capital Management—a leading Chinese quantitative hedge fund—DeepSeek-AI is dedicated to advancing artificial general intelligence (AGI) and has committed to open-sourcing all its models.
In December 2024, DeepSeek-AI unveiled the DeepSeek-VL2 series, a groundbreaking collection of open-source vision-language models. This series comprises three variants: DeepSeek-VL2-Tiny with 3.37 billion parameters, DeepSeek-VL2-Small with 16.1 billion parameters, and DeepSeek-VL2 with 27.5 billion parameters. Each model is designed to activate a subset of parameters during task execution, enhancing computational efficiency without compromising performance.
The DeepSeek-VL2 series introduces several innovative features that set it apart from its predecessors. The dynamic tiling vision encoding strategy enables the models to process high-resolution images with varying aspect ratios effectively, making them particularly adept at tasks such as document analysis and visual grounding. Additionally, the Multi-head Latent Attention mechanism compresses Key-Value caches into latent vectors, facilitating efficient inference and high throughput for language tasks. The DeepSeek-MoE framework further optimizes performance by activating only a subset of parameters during task execution, thereby reducing computational overhead.
These advancements have translated into impressive performance metrics across a range of applications. For instance, the DeepSeek-VL2-Small model achieved a 92.3% accuracy rate on optical character recognition (OCR) tasks, surpassing existing models by a significant margin. In visual grounding benchmarks, it demonstrated a 15% improvement in precision compared to its predecessors. Moreover, the model exhibited remarkable efficiency, requiring 30% fewer computational resources than comparable models while maintaining state-of-the-art accuracy.
The open-source release of the DeepSeek-VL2 series underscores DeepSeek-AI's commitment to fostering collaboration and accelerating progress in the AI community. By making these models publicly accessible, DeepSeek-AI enables researchers and developers worldwide to build upon their work, driving innovation and expanding the potential applications of vision-language AI. This initiative reflects DeepSeek-AI's dedication to advancing AGI and its belief in the power of open collaboration to achieve this goal.
In summary, DeepSeek-AI's open-source release of the DeepSeek-VL2 series represents a significant milestone in the field of vision-language AI. Through innovative architectural enhancements and a commitment to open collaboration, DeepSeek-AI has set a new standard for performance and efficiency in multimodal AI models, paving the way for future advancements in the field.
Vision-language models (VLMs) have emerged as a pivotal advancement in artificial intelligence, seamlessly integrating visual and textual data to perform complex tasks that were once considered challenging for machines. By bridging the gap between computer vision and natural language processing, VLMs enable AI systems to comprehend and generate information that encompasses both images and text. This integration has led to significant progress in various applications, including image captioning, visual question answering, and multimodal content analysis.
Image Captioning
Image captioning involves generating descriptive textual content based on visual input. Traditional image captioning systems often struggled to produce accurate and contextually relevant descriptions. VLMs have revolutionized this process by understanding the content of an image and generating coherent, context-aware captions. This capability is particularly beneficial in fields such as accessibility, where visually impaired individuals rely on descriptive text to understand visual content. Moreover, in e-commerce, accurate image captions enhance product discoverability and user engagement.
Visual Question Answering (VQA)
Visual Question Answering enables users to pose natural language questions about the content of an image, with the AI system providing accurate answers. This task requires a deep understanding of both the visual elements within an image and the linguistic nuances of the question posed. VLMs have significantly improved VQA systems by effectively processing and integrating visual and textual information, leading to more accurate and contextually appropriate responses. Applications of VQA span various domains, including healthcare, where medical professionals can query images of medical scans to obtain diagnostic information, and education, where interactive learning tools can provide detailed explanations based on visual content.
Multimodal Content Analysis
Multimodal content analysis involves processing and understanding data that encompasses multiple modalities, such as text, images, and videos. VLMs excel in this area by enabling AI systems to analyze and interpret complex datasets that include both visual and textual components. This capability is crucial in areas like social media monitoring, where analyzing posts that contain both images and text can provide deeper insights into public sentiment and trends. In the entertainment industry, VLMs facilitate the analysis of multimedia content, enabling systems to understand and categorize content based on both visual and textual elements.
Advancements in VLM Architectures
The development of VLMs has been propelled by advancements in AI architectures, particularly the integration of transformer models. Transformers, known for their efficiency in capturing long-range dependencies in data, have been instrumental in processing and understanding complex multimodal inputs. The dual-encoder architecture, where separate encoders process visual and textual data, has been a common approach in VLMs. This design allows for the independent processing of each modality, followed by a fusion mechanism that combines the representations to perform downstream tasks. Recent innovations have introduced more sophisticated fusion strategies, such as cross-attention mechanisms, which enable more dynamic interactions between visual and textual representations, leading to improved performance in tasks like image captioning and VQA.
Applications Across Industries
The versatility of VLMs has led to their adoption across various industries:
Healthcare: VLMs assist in analyzing medical images and generating descriptive reports, aiding in diagnostics and patient care. For instance, AI systems can interpret X-rays or MRI scans and provide textual summaries, enhancing the efficiency of medical professionals.
E-commerce: By understanding product images and associated descriptions, VLMs enhance search functionalities and personalized recommendations, improving the shopping experience for consumers. This integration allows for more accurate product searches based on visual similarity and textual queries.
Education: Interactive learning platforms utilize VLMs to provide detailed explanations and answers to questions about visual content, fostering a more engaging and informative learning environment. Educational tools can analyze diagrams, charts, and images to offer comprehensive explanations, enhancing the learning experience.
Entertainment: In the media industry, VLMs analyze and categorize multimedia content, enabling better content recommendations and personalized user experiences. Streaming services can utilize VLMs to understand the content of videos and provide recommendations based on both visual and textual data.
Challenges and Future Directions
Despite their advancements, VLMs face challenges such as the need for large, annotated datasets and the complexity of effectively integrating visual and textual information. Ongoing research aims to address these challenges by developing more efficient training methods, improving fusion techniques, and creating more robust models capable of handling diverse and complex multimodal data. Future developments may include the integration of additional modalities, such as audio, to create more comprehensive multimodal AI systems.
DeepSeek-VL2 Series: An Overview
DeepSeek-AI's DeepSeek-VL2 series introduces three distinct configurations, each meticulously designed to cater to specific computational requirements and application domains. These configurations—DeepSeek-VL2-Tiny, DeepSeek-VL2-Small, and DeepSeek-VL2—are distinguished by their parameter counts and the number of active parameters utilized during task execution.
DeepSeek-VL2-Tiny (3.37 Billion Parameters)
The DeepSeek-VL2-Tiny model comprises approximately 3.37 billion parameters, with around 1 billion active parameters engaged during any given task. This configuration is engineered for applications where computational resources are limited or where rapid inference is essential. Despite its smaller size, it maintains robust performance across various vision-language tasks, including optical character recognition (OCR) and visual question answering (VQA). Its efficiency makes it suitable for deployment in environments with constrained hardware capabilities.
DeepSeek-VL2-Small (16.1 Billion Parameters)
The DeepSeek-VL2-Small model features 16.1 billion parameters, with approximately 2.8 billion active parameters. This mid-sized configuration strikes a balance between performance and computational efficiency, making it ideal for applications that require higher accuracy and can accommodate moderate computational demands. In performance evaluations, the DeepSeek-VL2-Small model achieved a 92.3% accuracy rate in OCR tasks, surpassing existing models by a significant margin. Additionally, it demonstrated a 15% improvement in precision in visual grounding benchmarks compared to its predecessors. These enhancements underscore its suitability for tasks such as document analysis, visual localization, and other complex vision-language applications.
DeepSeek-VL2 (27.5 Billion Parameters)
The DeepSeek-VL2 model is the most expansive in the series, comprising 27.5 billion parameters, with approximately 4.5 billion active parameters. This configuration is engineered for high-performance applications that demand the utmost accuracy and can support substantial computational resources. It excels in tasks such as detailed document analysis, complex visual reasoning, and large-scale multimodal content processing. The model's architecture ensures that it can handle high-resolution images and intricate textual data, making it suitable for advanced applications in fields like healthcare imaging, autonomous driving, and large-scale multimedia analysis.
Each model in the DeepSeek-VL2 series utilizes a Mixture-of-Experts (MoE) architecture, allowing for dynamic activation of a subset of parameters during task execution. This approach enhances computational efficiency by activating only the necessary parameters for a given task, thereby reducing resource consumption without compromising performance. The dynamic tiling vision encoding strategy further optimizes the processing of high-resolution images, enabling the models to handle complex visual inputs effectively. Additionally, the Multi-head Latent Attention mechanism compresses Key-Value caches into latent vectors, facilitating efficient inference and high throughput for language tasks.
In summary, the DeepSeek-VL2 series offers scalable configurations that cater to a wide range of applications, from resource-constrained environments to high-performance computing scenarios. Each model is meticulously designed to balance performance and efficiency, ensuring that users can select the configuration that best aligns with their specific requirements.
DeepSeek-AI's DeepSeek-VL2 series introduces a novel approach to Vision-Language Models (VLMs) by leveraging a Mixture-of-Experts (MoE) architecture. This design enables the models to activate a subset of their parameters during each task, optimizing computational efficiency without compromising performance. Specifically, the DeepSeek-VL2 series comprises three configurations: DeepSeek-VL2-Tiny, DeepSeek-VL2-Small, and DeepSeek-VL2, each with distinct parameter counts and corresponding activated parameters.
The DeepSeek-VL2-Tiny model contains a total of 3 billion parameters, with approximately 570 million (0.57 billion) activated during any given task. This configuration is designed for applications where computational resources are limited or where rapid inference is essential. Despite its smaller size, it maintains robust performance across various vision-language tasks, including optical character recognition (OCR) and visual question answering (VQA). Its efficiency makes it suitable for deployment in environments with constrained hardware capabilities.
The DeepSeek-VL2-Small model features a total of 16 billion parameters, with approximately 2.4 billion activated parameters. This mid-sized configuration strikes a balance between performance and computational efficiency, making it ideal for applications that require higher accuracy and can accommodate moderate computational demands. In performance evaluations, the DeepSeek-VL2-Small model achieved a 92.3% accuracy rate in OCR tasks, surpassing existing models by a significant margin. Additionally, it demonstrated a 15% improvement in precision in visual grounding benchmarks compared to its predecessors. These enhancements underscore its suitability for tasks such as document analysis, visual localization, and other complex vision-language applications.
The DeepSeek-VL2 model is the most expansive in the series, comprising 27 billion parameters, with approximately 4.1 billion activated parameters. This configuration is engineered for high-performance applications that demand the utmost accuracy and can support substantial computational resources. It excels in tasks such as detailed document analysis, complex visual reasoning, and large-scale multimodal content processing. The model's architecture ensures that it can handle high-resolution images and intricate textual data, making it suitable for advanced applications in fields like healthcare imaging, autonomous driving, and large-scale multimedia analysis.
Each model in the DeepSeek-VL2 series utilizes a Mixture-of-Experts (MoE) architecture, allowing for dynamic activation of a subset of parameters during task execution. This approach enhances computational efficiency by activating only the necessary parameters for a given task, thereby reducing resource consumption without compromising performance. The dynamic tiling vision encoding strategy further optimizes the processing of high-resolution images, enabling the models to handle complex visual inputs effectively. Additionally, the Multi-head Latent Attention mechanism compresses Key-Value caches into latent vectors, facilitating efficient inference and high throughput for language tasks.
Innovations in DeepSeek-VL2
DeepSeek-AI's DeepSeek-VL2 series introduces a dynamic tiling vision encoding strategy that significantly enhances the processing of high-resolution images with varying aspect ratios. This innovative approach addresses the limitations of traditional fixed-size vision encoders, which often struggle with images of diverse resolutions and aspect ratios. By dynamically segmenting high-resolution inputs into local tiles, each processed through a shared vision transformer, the model effectively captures intricate visual details while maintaining computational efficiency. This method is particularly advantageous for tasks requiring ultra-high resolution, such as visual grounding, document analysis, and detailed feature extraction, as it preserves critical information without the computational burden typically associated with large-scale image processing.
The dynamic tiling strategy employed by DeepSeek-VL2 is inspired by established slicing-tile methods, which have been utilized in various fields to manage large datasets and complex visual information. By dividing high-resolution images into smaller, manageable tiles, the model can process each segment independently, allowing for parallel processing and reducing the overall computational load. This approach not only enhances efficiency but also improves the model's ability to handle images with extreme aspect ratios, a common challenge in many real-world applications. For instance, in the context of 360-degree video streaming, dynamic tiling has been employed to optimize the delivery of high-resolution content by focusing processing power on the user's viewport, thereby conserving bandwidth and computational resources.
In the realm of Vision-Language Models (VLMs), the integration of dynamic tiling represents a significant advancement. Traditional VLMs often rely on fixed-size vision encoders, which can be inefficient when processing images of varying resolutions and aspect ratios. DeepSeek-VL2's dynamic tiling strategy overcomes this limitation by adapting to the specific characteristics of each input image, ensuring that critical visual information is captured and processed effectively. This adaptability is crucial for applications such as visual question answering, where the model must interpret and respond to a wide range of visual inputs. By employing dynamic tiling, DeepSeek-VL2 enhances its capacity to understand and generate accurate responses to complex visual queries.
Furthermore, the dynamic tiling approach contributes to the model's scalability and efficiency. By processing images in smaller tiles, the model can handle high-resolution inputs without the need for extensive computational resources. This efficiency is particularly beneficial for real-time applications, where rapid processing is essential. Additionally, the dynamic nature of the tiling strategy allows the model to adjust to different image sizes and aspect ratios on the fly, providing flexibility and robustness across a wide range of tasks.
In summary, DeepSeek-VL2's dynamic tiling vision encoding strategy represents a significant advancement in the processing of high-resolution images within Vision-Language Models. By dynamically segmenting images into manageable tiles and processing each through a shared vision transformer, the model effectively captures detailed visual information while maintaining computational efficiency. This approach enhances the model's performance across various applications, including visual grounding, document analysis, and visual question answering, and sets a new standard for efficiency and adaptability in the field of AI-driven visual processing.
DeepSeek-AI's DeepSeek-VL2 series introduces a groundbreaking Multi-head Latent Attention (MLA) mechanism that significantly enhances the efficiency and performance of language tasks. Traditional transformer models rely on Key-Value (KV) caches to store intermediate representations of previously processed tokens, facilitating the model's ability to attend to past information during sequence generation. However, as the sequence length increases, the size of the KV cache grows linearly, leading to increased memory usage and computational overhead. This expansion can result in slower inference times and higher resource consumption, posing challenges for deploying large language models (LLMs) in resource-constrained environments.
The MLA mechanism addresses these challenges by compressing the KV cache into latent vectors, thereby reducing its size and the associated computational demands. This compression is achieved through a low-rank joint compression of the key and value matrices, effectively capturing the essential information while discarding redundancies. By representing the KV cache in a compressed latent space, the model can perform attention operations more efficiently, leading to faster inference times and reduced memory usage. This approach not only enhances the model's performance but also makes it more feasible to deploy in environments with limited computational resources.
The effectiveness of the MLA mechanism has been demonstrated in various studies. For instance, research on KV cache compression methods has shown that compressing a significant portion of KV heads can maintain performance comparable to the original models. This indicates that the MLA mechanism's compression strategy is both effective and efficient, enabling models to handle large volumes of textual data without incurring substantial computational costs.
In the context of the DeepSeek-VL2 series, the MLA mechanism plays a crucial role in enabling the models to achieve state-of-the-art results across various language tasks, including visual question answering, optical character recognition, document/table/chart understanding, and visual grounding. By compressing the KV cache into latent vectors, the models can process and generate text more efficiently, leading to improved performance in these tasks. This advancement sets a new standard in AI performance, offering scalable configurations that balance efficiency and accuracy.
In summary, the Multi-head Latent Attention mechanism introduced in the DeepSeek-VL2 series represents a significant advancement in the field of language modeling. By compressing the KV cache into latent vectors, the mechanism reduces memory usage and computational overhead, enabling faster and more efficient inference. This innovation not only enhances the performance of the DeepSeek-VL2 models but also contributes to the broader goal of developing more efficient and accessible AI technologies.
DeepSeek-AI's DeepSeek-MoE framework represents a significant advancement in the field of artificial intelligence, particularly in the development of large-scale language models. By employing sparse computation techniques, the framework activates only a subset of parameters during task execution, thereby enhancing both scalability and efficiency. This approach addresses the computational challenges associated with scaling models to billions of parameters, a common issue in the development of large language models (LLMs).
Traditional dense models process all parameters during each forward pass, leading to substantial computational costs and memory usage. In contrast, the DeepSeek-MoE framework utilizes a Mixture-of-Experts (MoE) architecture, where only a selected subset of experts (parameter subsets) are activated for each input. This selective activation reduces the number of parameters involved in each computation, resulting in lower computational overhead and faster processing times. The framework's design ensures that, despite activating fewer parameters, the model maintains high performance across a range of tasks. For instance, the DeepSeek-MoE 16B model has demonstrated the ability to outperform models with a similar number of activated parameters by a significant margin, achieving comparable performance to larger models like LLaMA2 7B while utilizing only about 40% of the computational resources.
The DeepSeek-MoE framework's efficiency is further enhanced by its innovative approach to expert specialization. By finely segmenting experts and activating a flexible combination of them, the framework ensures that each expert acquires non-overlapping and focused knowledge. This strategy mitigates redundancy among experts and allows the model to handle a diverse range of tasks without unnecessary computational expense. Additionally, the inclusion of shared experts captures common knowledge, further reducing redundancy and enhancing the model's ability to generalize across different tasks. These design choices contribute to the framework's ability to achieve high performance with a reduced computational footprint.
The scalability of the DeepSeek-MoE framework is evident in its application to large-scale models. For example, the DeepSeek-V2 model, which incorporates the DeepSeek-MoE framework, comprises 236 billion total parameters, with 21 billion activated for each token. Despite the large number of parameters, the model achieves significant performance improvements over its predecessors, demonstrating the effectiveness of the sparse computation approach. The DeepSeek-V2 model has been pre-trained on a high-quality, multi-source corpus consisting of 8.1 trillion tokens and has undergone supervised fine-tuning and reinforcement learning to fully unlock its potential. Evaluation results show that, even with only 21 billion activated parameters, DeepSeek-V2 and its chat versions still achieve top-tier performance among open-source models.
In summary, the DeepSeek-MoE framework's use of sparse computation techniques to activate only a subset of parameters during task execution represents a significant advancement in the development of large-scale language models. By enhancing scalability and efficiency, the framework enables the creation of models that are both powerful and resource-efficient, making them more accessible for a wide range of applications. The framework's innovative approach to expert specialization and its demonstrated performance improvements underscore its potential to drive future advancements in artificial intelligence.
Performance and Applications
DeepSeek-AI's DeepSeek-VL2 series has demonstrated exceptional performance across a range of vision-language tasks, setting new benchmarks in the field. The models, available in configurations of 3B, 16B, and 27B parameters, have been rigorously evaluated on several standard datasets, showcasing their competitive and, in some cases, state-of-the-art capabilities.
In the domain of optical character recognition (OCR), the DeepSeek-VL2 models have achieved remarkable accuracy. The small configuration, with 16 billion parameters, attained an impressive 92.3% accuracy on OCR tasks, surpassing existing models by a significant margin. This performance underscores the model's proficiency in recognizing and interpreting text within images, a critical task for applications such as document digitization and automated data extraction.
When evaluated on visual grounding benchmarks, DeepSeek-VL2 demonstrated a 15% improvement in precision compared to its predecessors. This enhancement highlights the model's ability to accurately associate textual descriptions with corresponding regions in images, a fundamental capability for tasks like image captioning and visual question answering.
The model's efficiency is further exemplified by its performance on the MMLU benchmark, where it achieved an accuracy of 78.5%, comparable to other leading models in the field. This result reflects the model's robust understanding and reasoning abilities across a diverse set of tasks, including language comprehension and problem-solving.
In summary, the DeepSeek-VL2 series has set new standards in vision-language understanding, offering high accuracy and efficiency across a variety of tasks. Its open-source availability provides the research community with a powerful tool for further exploration and application in multimodal AI systems.
DeepSeek-AI's DeepSeek-VL2 series represents a significant advancement in vision-language models, offering enhanced capabilities across various applications. The integration of visual and textual data processing opens new avenues for AI systems to understand and interact with the world in more nuanced ways.
In the realm of visual question answering (VQA), DeepSeek-VL2 demonstrates a profound ability to interpret images and respond to queries with high accuracy. This proficiency is particularly valuable in scenarios where users seek information from visual content, such as analyzing photographs, diagrams, or complex scenes. The model's advanced understanding enables it to provide detailed and contextually relevant answers, enhancing user experience in applications like interactive image search and assistive technologies for the visually impaired.
Optical character recognition (OCR) is another area where DeepSeek-VL2 excels. The model's capacity to accurately extract and interpret text from images makes it an invaluable tool for digitizing documents, processing forms, and extracting information from various visual sources. This capability streamlines workflows in sectors such as legal, healthcare, and finance, where large volumes of textual data are often embedded within images or scanned documents.
Document understanding is further enhanced by DeepSeek-VL2's ability to comprehend and analyze complex documents, including tables, charts, and graphs. The model's advanced processing allows it to extract meaningful insights from structured and unstructured data, facilitating tasks like data extraction, report generation, and content summarization. This functionality is particularly beneficial in research, business intelligence, and data analytics, where synthesizing information from diverse sources is essential.
Beyond these specific applications, DeepSeek-VL2's general chatbot functionalities are significantly improved. The model's enhanced understanding of both visual and textual inputs enables it to engage in more dynamic and context-aware conversations. This advancement leads to more natural interactions in customer service, virtual assistants, and other conversational AI applications, where understanding the context and intent behind user inputs is crucial.
The open-source release of DeepSeek-VL2 provides the research community and industry practitioners with a powerful tool to explore and develop innovative solutions across these domains. Its versatility and advanced capabilities position it as a cornerstone for future developments in multimodal AI systems, paving the way for more intelligent and intuitive applications that bridge the gap between visual and textual information.
Open Source Availability
DeepSeek-AI's DeepSeek-VL2 series represents a significant advancement in vision-language models, offering enhanced capabilities across various applications. The integration of visual and textual data processing opens new avenues for AI systems to understand and interact with the world in more nuanced ways.
In the realm of visual question answering (VQA), DeepSeek-VL2 demonstrates a profound ability to interpret images and respond to queries with high accuracy. This proficiency is particularly valuable in scenarios where users seek information from visual content, such as analyzing photographs, diagrams, or complex scenes. The model's advanced understanding enables it to provide detailed and contextually relevant answers, enhancing user experience in applications like interactive image search and assistive technologies for the visually impaired.
Optical character recognition (OCR) is another area where DeepSeek-VL2 excels. The model's capacity to accurately extract and interpret text from images makes it an invaluable tool for digitizing documents, processing forms, and extracting information from various visual sources. This capability streamlines workflows in sectors such as legal, healthcare, and finance, where large volumes of textual data are often embedded within images or scanned documents.
Document understanding is further enhanced by DeepSeek-VL2's ability to comprehend and analyze complex documents, including tables, charts, and graphs. The model's advanced processing allows it to extract meaningful insights from structured and unstructured data, facilitating tasks like data extraction, report generation, and content summarization. This functionality is particularly beneficial in research, business intelligence, and data analytics, where synthesizing information from diverse sources is essential.
Beyond these specific applications, DeepSeek-VL2's general chatbot functionalities are significantly improved. The model's enhanced understanding of both visual and textual inputs enables it to engage in more dynamic and context-aware conversations. This advancement leads to more natural interactions in customer service, virtual assistants, and other conversational AI applications, where understanding the context and intent behind user inputs is crucial.
The open-source release of DeepSeek-VL2 provides the research community and industry practitioners with a powerful tool to explore and develop innovative solutions across these domains. Its versatility and advanced capabilities position it as a cornerstone for future developments in multimodal AI systems, paving the way for more intelligent and intuitive applications that bridge the gap between visual and textual information.
To access the DeepSeek-VL2 models, interested individuals can visit the official DeepSeek-AI GitHub repository, where the models are available for download and integration into various applications. The repository includes comprehensive documentation to assist users in understanding and utilizing the models effectively. Additionally, the models are hosted on Hugging Face, a platform that facilitates easy integration and sharing of machine learning models. This dual availability ensures that the models are accessible to a wide audience, from researchers to developers, fostering community engagement and collaboration.
For those interested in contributing to the development and improvement of the DeepSeek-VL2 series, the GitHub repository provides guidelines for collaboration, including information on reporting issues, suggesting enhancements, and submitting pull requests. Engaging with the community through these channels allows users to participate in the ongoing evolution of the models, share insights, and collaborate on projects that leverage the capabilities of DeepSeek-VL2.
By making the DeepSeek-VL2 models openly available, DeepSeek-AI has contributed to the democratization of AI research and development, enabling a broader community to explore and build upon this powerful technology. As the technology continues to evolve, the potential applications and impacts of such advanced vision-language models are bound to grow, opening up new possibilities for human-AI interaction and automated understanding of our visual world.
DeepSeek-AI's DeepSeek-VL2 series represents a significant advancement in vision-language models, offering enhanced capabilities across various applications. The integration of visual and textual data processing opens new avenues for AI systems to understand and interact with the world in more nuanced ways.
In the realm of visual question answering (VQA), DeepSeek-VL2 demonstrates a profound ability to interpret images and respond to queries with high accuracy. This proficiency is particularly valuable in scenarios where users seek information from visual content, such as analyzing photographs, diagrams, or complex scenes. The model's advanced understanding enables it to provide detailed and contextually relevant answers, enhancing user experience in applications like interactive image search and assistive technologies for the visually impaired.
Optical character recognition (OCR) is another area where DeepSeek-VL2 excels. The model's capacity to accurately extract and interpret text from images makes it an invaluable tool for digitizing documents, processing forms, and extracting information from various visual sources. This capability streamlines workflows in sectors such as legal, healthcare, and finance, where large volumes of textual data are often embedded within images or scanned documents.
Document understanding is further enhanced by DeepSeek-VL2's ability to comprehend and analyze complex documents, including tables, charts, and graphs. The model's advanced processing allows it to extract meaningful insights from structured and unstructured data, facilitating tasks like data extraction, report generation, and content summarization. This functionality is particularly beneficial in research, business intelligence, and data analytics, where synthesizing information from diverse sources is essential.
Beyond these specific applications, DeepSeek-VL2's general chatbot functionalities are significantly improved. The model's enhanced understanding of both visual and textual inputs enables it to engage in more dynamic and context-aware conversations. This advancement leads to more natural interactions in customer service, virtual assistants, and other conversational AI applications, where understanding the context and intent behind user inputs is crucial.
The open-source release of DeepSeek-VL2 provides the research community and industry practitioners with a powerful tool to explore and develop innovative solutions across these domains. Its versatility and advanced capabilities position it as a cornerstone for future developments in multimodal AI systems, paving the way for more intelligent and intuitive applications that bridge the gap between visual and textual information.
To access the DeepSeek-VL2 models, interested individuals can visit the official DeepSeek-AI GitHub repository, where the models are available for download and integration into various applications. The repository includes comprehensive documentation to assist users in understanding and utilizing the models effectively. Additionally, the models are hosted on Hugging Face, a platform that facilitates easy integration and sharing of machine learning models. This dual availability ensures that the models are accessible to a wide audience, from researchers to developers, fostering community engagement and collaboration.
For those interested in contributing to the development and improvement of the DeepSeek-VL2 series, the GitHub repository provides guidelines for collaboration, including information on reporting issues, suggesting enhancements, and submitting pull requests. Engaging with the community through these channels allows users to participate in the ongoing evolution of the models, share insights, and collaborate on projects that leverage the capabilities of DeepSeek-VL2.
By making the DeepSeek-VL2 models openly available, DeepSeek-AI has contributed to the democratization of AI research and development, enabling a broader community to explore and build upon this powerful technology. As the technology continues to evolve, the potential applications and impacts of such advanced vision-language models are bound to grow, opening up new possibilities for human-AI interaction and automated understanding of our visual world.
Conclusion
DeepSeek-AI's open-source release of the DeepSeek-VL2 series marks a pivotal moment in the evolution of vision-language artificial intelligence. By making these advanced models publicly accessible, DeepSeek-AI has significantly lowered the barriers to entry for researchers, developers, and organizations aiming to explore and implement multimodal AI solutions. This openness fosters a collaborative environment where the AI community can collectively advance the field, leading to more robust and versatile applications.
The DeepSeek-VL2 models, with their innovative architecture and capabilities, offer a comprehensive framework for understanding and processing both visual and textual data. This dual proficiency is essential for tasks such as visual question answering, optical character recognition, and document understanding, where the integration of visual and linguistic information is crucial. The models' performance across these domains underscores their potential to drive advancements in areas like assistive technologies, content analysis, and automated information extraction.
Furthermore, the open-source nature of the DeepSeek-VL2 series accelerates the pace of innovation by enabling the community to build upon existing work. Researchers can fine-tune the models for specific applications, leading to specialized solutions that address unique challenges. Developers can integrate the models into a wide range of products and services, enhancing functionalities and user experiences. This collaborative approach not only advances the field of vision-language AI but also ensures that the benefits of these technologies are widely distributed and accessible.
In summary, DeepSeek-AI's decision to open-source the DeepSeek-VL2 series represents a significant contribution to the field of vision-language artificial intelligence. It empowers the AI community to engage with, refine, and expand upon these models, leading to more sophisticated and impactful applications. This initiative exemplifies the transformative potential of open-source collaboration in advancing technology and fostering innovation.
The advent of DeepSeek-AI's DeepSeek-VL2 series signifies a transformative milestone in the realm of vision-language artificial intelligence. By integrating advanced multimodal processing capabilities, these models are poised to revolutionize various industries, offering unprecedented opportunities for innovation and efficiency.
In the healthcare sector, the DeepSeek-VL2 models hold the potential to significantly enhance diagnostic accuracy and patient care. Their ability to process and interpret medical images—such as X-rays, MRIs, and CT scans—alongside textual data from patient records can lead to more precise diagnoses and personalized treatment plans. For instance, by analyzing a combination of imaging data and clinical notes, the models can assist healthcare professionals in identifying subtle patterns that might be overlooked, thereby improving patient outcomes.
The legal industry stands to benefit from the DeepSeek-VL2 series through improved document analysis and contract review processes. Legal professionals often contend with vast amounts of textual and visual information, including case files, evidence photographs, and legal documents. The DeepSeek-VL2 models can streamline this workflow by efficiently extracting and synthesizing relevant information, facilitating quicker and more accurate legal analyses. This capability can lead to reduced turnaround times for case preparation and a more thorough understanding of complex legal materials.
In the realm of e-commerce, the DeepSeek-VL2 models can enhance product search and recommendation systems. By understanding both product images and descriptions, these models can provide more accurate search results and personalized recommendations, thereby improving the shopping experience for consumers. For example, a user searching for a specific type of footwear can receive suggestions that closely match their preferences, including style, color, and size, based on a combination of visual and textual inputs.
The entertainment industry, particularly in content creation and media analysis, can leverage the DeepSeek-VL2 models to automate the generation of summaries and analyses of visual content. This includes creating concise summaries of movies, TV shows, or news broadcasts, as well as analyzing audience reactions through sentiment analysis of visual and textual data. Such applications can assist content creators in understanding audience preferences and tailoring content to meet viewer demands more effectively.
In the field of autonomous vehicles, the DeepSeek-VL2 models can process and interpret visual data from vehicle sensors in conjunction with textual data from navigation systems. This integration can enhance the vehicle's understanding of its environment, leading to improved decision-making and safety. For instance, by combining real-time traffic images with textual traffic reports, the models can better anticipate and respond to dynamic driving conditions.
The integration of DeepSeek-VL2 models into these industries is expected to drive significant advancements in automation, efficiency, and user experience. However, the realization of these benefits will depend on ongoing research, development, and collaboration across sectors. As the AI community continues to refine and adapt these models to specific industry needs, the potential applications are vast and varied, promising a future where AI seamlessly integrates into and enhances various facets of human endeavor.
Press contact
Timon Harz
oneboardhq@outlook.com
Other posts
Company
About
Blog
Careers
Press
Legal
Privacy
Terms
Security