Timon Harz
December 20, 2024
Advancing Clinical Decision Support: Analyzing OpenAI’s O1-Preview Model for Medical Reasoning Capabilities
Explore the limitations of traditional benchmarks in evaluating large language models for medical tasks and how OpenAI’s o1-preview model enhances clinical decision-making.
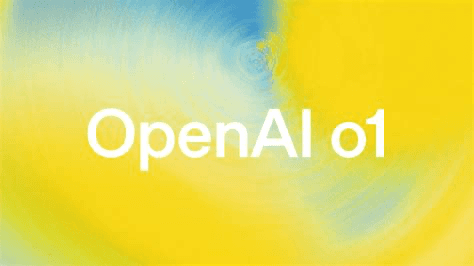
The evaluation of large language models (LLMs) in medical tasks has traditionally been based on multiple-choice question benchmarks. However, these benchmarks often fail to capture the complexity of clinical decision-making and tend to produce saturated results with high performance from LLMs. They do not accurately reflect the real-world challenges physicians face in clinical scenarios. Clinical reasoning, which involves the cognitive process of analyzing and synthesizing medical data for diagnosis and treatment, offers a more meaningful benchmark for assessing model performance. Recent LLMs have shown the ability to outperform clinicians in both routine and complex diagnostic tasks, surpassing older AI tools that relied on regression models, Bayesian approaches, and rule-based systems.
Recent advancements in LLMs, particularly foundation models, have demonstrated exceptional performance in diagnostic benchmarks, with techniques such as Chain-of-Thought (CoT) prompting further enhancing their reasoning abilities. OpenAI’s o1-preview model, launched in September 2024, incorporates a native CoT mechanism that improves its reasoning during complex problem-solving tasks. This model has surpassed GPT-4 in addressing intricate challenges such as informatics and medicine. Despite these advancements, traditional multiple-choice benchmarks fail to capture the nuanced nature of clinical decision-making. These benchmarks allow models to exploit semantic patterns rather than engaging in true reasoning. Real-world clinical practice requires multi-step, dynamic reasoning, where models must continuously process and integrate diverse data sources, refine differential diagnoses, and make critical decisions under uncertainty.
A study conducted by researchers from institutions like Beth Israel Deaconess Medical Center, Stanford University, and Harvard Medical School evaluated OpenAI’s o1-preview model. The model, designed to enhance reasoning through chain-of-thought processes, was tested on five key tasks: differential diagnosis generation, reasoning explanation, triage diagnosis, probabilistic reasoning, and management reasoning. Expert physicians assessed the model’s performance against prior LLMs and human benchmarks using validated metrics. The results showed significant improvements in diagnostic and management reasoning, but no advancements in probabilistic reasoning or triage. This highlights the need for robust benchmarks and real-world trials to evaluate LLMs in clinical settings.
The study assessed o1-preview’s diagnostic capabilities using diverse medical cases, such as NEJM Clinicopathologic Conference (CPC) cases, NEJM Healer cases, Grey Matters management cases, landmark diagnostic cases, and probabilistic reasoning tasks. Outcomes focused on the quality of differential diagnoses, testing plans, clinical reasoning documentation, and identifying critical diagnoses. Physicians evaluated the model using metrics like Bond Scores, R-IDEA, and normalized rubrics. Statistical analyses, including McNemar’s test and mixed-effects models, were conducted in R. The results revealed that o1-preview excelled in reasoning tasks but highlighted areas for improvement, particularly in probabilistic reasoning.
The evaluation of o1-preview’s diagnostic capabilities using NEJM cases demonstrated its strengths, as it correctly included the diagnosis in 78.3% of cases, outperforming GPT-4 (88.6% vs. 72.9%). It achieved high accuracy in test selection (87.5%) and performed excellently in clinical reasoning, scoring 78/80 on the R-IDEA scale for NEJM Healer cases, surpassing both GPT-4 and physicians. In management vignettes, o1-preview outperformed both GPT-4 and physicians by over 40%. It also achieved a median score of 97% in landmark diagnostic cases, comparable to GPT-4 but higher than physicians. While its performance in probabilistic reasoning was similar to GPT-4, o1-preview performed better in coronary stress test accuracy.
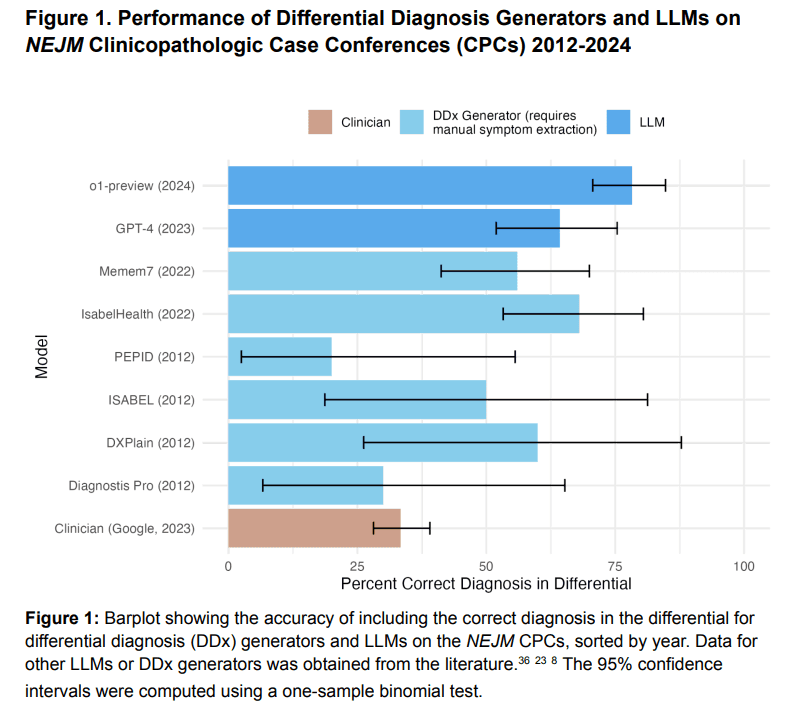
In conclusion, the o1-preview model outperformed both GPT-4 and human baselines in medical reasoning across five key tasks, including differential diagnosis, diagnostic reasoning, and management decisions. However, it showed no significant advancements over GPT-4 in areas such as probabilistic reasoning and critical diagnosis identification. These results underscore the potential of LLMs in clinical decision support, though further real-world trials are essential to assess their suitability for patient care. As current benchmarks like NEJM CPCs approach saturation, there is a clear need for more challenging and realistic evaluations. Additionally, limitations such as verbosity, the lack of studies on human-computer interaction, and a focus on internal medicine highlight the importance of broader, more comprehensive assessments.
Press contact
Timon Harz
oneboardhq@outlook.com
Other posts
Company
About
Blog
Careers
Press
Legal
Privacy
Terms
Security